Pose calculation method and system combining deep learning and geometric optimization
A technology of geometric optimization and deep learning, applied in the field of visual odometry in the field of computer vision, can solve problems such as weak interpretability and generalization ability, deviation of the true value of the result of pose estimation, ignoring the geometric constraints of dependencies, etc. Achieve the effect of strong interpretability and generalization ability, and improve the accuracy of pose estimation
- Summary
- Abstract
- Description
- Claims
- Application Information
AI Technical Summary
Problems solved by technology
Method used
Image
Examples
Embodiment 1
[0041] Such as Figure 1-6 As shown, a pose calculation method and system combining deep learning and geometric optimization, including pose estimation module PoseNet and depth estimation module DepthNet, PoseNet module and Depth module need pre-training, pre-training uses existing models, for example, you can use In the pre-training model on KITTI odometry sequence 00-08, the PoseNet module inputs a binocular video sequence with a video frame length of 3, a video resolution of 1024×320, and outputs a 6DoF relative pose transformation, which is converted into =SE(3 ) (SE(3) is a special Euclidean group in Lie algebra); the DepthNet module inputs a single video frame and outputs a single channel depth map. The structure of PoseNet is a convolutional neural network structure. For example, a 7-layer convolutional layer is used. After each convolutional layer, an activation function is connected. The convolution kernel sizes are 7, 5, 3, 3, 3, 3, and 3 respectively. The structure...
PUM
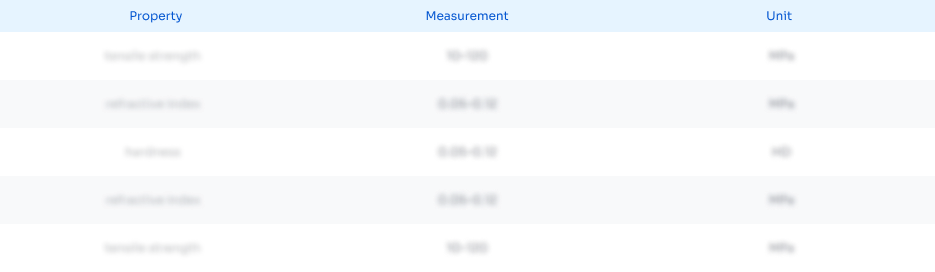
Abstract
Description
Claims
Application Information
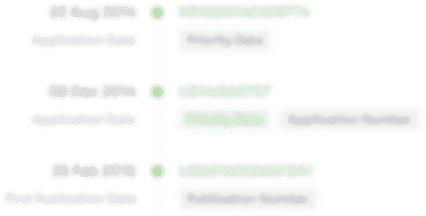
- R&D
- Intellectual Property
- Life Sciences
- Materials
- Tech Scout
- Unparalleled Data Quality
- Higher Quality Content
- 60% Fewer Hallucinations
Browse by: Latest US Patents, China's latest patents, Technical Efficacy Thesaurus, Application Domain, Technology Topic, Popular Technical Reports.
© 2025 PatSnap. All rights reserved.Legal|Privacy policy|Modern Slavery Act Transparency Statement|Sitemap|About US| Contact US: help@patsnap.com