Iterative 6D pose estimation method and device based on deep learning
A technology of pose estimation and deep learning, which is applied in the field of iterative 6D pose estimation based on deep learning, can solve the problems of RANSAC algorithm time-consuming, not reflecting pose estimation, PnP algorithm does not belong, etc., and achieves simple structure and fast running time. Reduce, improve the effect of accuracy
- Summary
- Abstract
- Description
- Claims
- Application Information
AI Technical Summary
Problems solved by technology
Method used
Image
Examples
Embodiment Construction
[0027] In order to make the technical solution of the present invention clearer, the specific implementation of the present invention will be described more fully below in conjunction with the accompanying drawings of the embodiments of the present invention. The described embodiments are only some, not all, embodiments of the present invention. Based on the embodiments of the present invention, all other embodiments obtained by persons of ordinary skill in the art without any creative work belong to the scope of the present invention.
[0028] The following describes the iterative 6D pose estimation method and device based on deep learning according to the embodiments of the present invention with reference to the accompanying drawings. figure 1 It is a schematic flowchart of an iterative 6D pose estimation method based on deep learning provided by an embodiment of the present invention. The iterative 6D pose estimation method based on deep learning in the embodiment of the ...
PUM
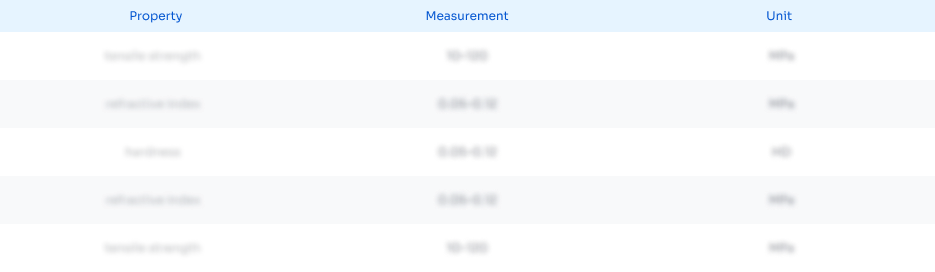
Abstract
Description
Claims
Application Information
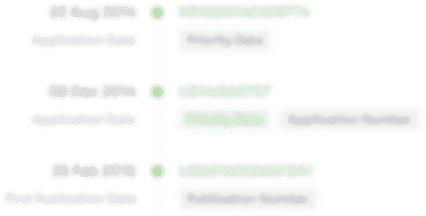
- R&D Engineer
- R&D Manager
- IP Professional
- Industry Leading Data Capabilities
- Powerful AI technology
- Patent DNA Extraction
Browse by: Latest US Patents, China's latest patents, Technical Efficacy Thesaurus, Application Domain, Technology Topic, Popular Technical Reports.
© 2024 PatSnap. All rights reserved.Legal|Privacy policy|Modern Slavery Act Transparency Statement|Sitemap|About US| Contact US: help@patsnap.com