Hyperspectral image denoising method based on non-convex low rank matrix decomposition
A technique of hyperspectral image, low rank matrix, applied in the field of hyperspectral image denoising
- Summary
- Abstract
- Description
- Claims
- Application Information
AI Technical Summary
Problems solved by technology
Method used
Image
Examples
Embodiment
[0147]The experimental hardware environment for this implementation is: Intel-Core4i76700 3.4GHz, 16G memory, graphics card NVIDIAGeForce GTX950. The software environment is MATLAB 2015b. The test images are derived from hyperspectral image datasets published on the Internet: Hyperspectral Digital Imagery Collection Experiment (HYDICE) urbandataset, the Earth Observing-1 (EO-1) Hyperion Australia dataset, and the Airborne Visible / InfraredImaging Spectrometer (AVIRIS) Indian Pines dataset. In order to verify the effectiveness of the method, the present invention has selected an advanced denoising method proposed in recent years: Video Block Matching 3-D filtering method (Video Block Matching 3-D filtering, VBM3D [1] ), low-rank matrix recovery method (Low-RankMatrix Recovery, LRMR [2] ), noise-adaptive iterative low-rank matrix approximation method (Noise-AdjustedIterative Low-Rank Matrix Approximation, NAILRMA [3] ), a weighted p-norm-based low-rank matrix approximation met...
example 1
[0148] Example 1: This example selects a typical data set in the field of remote sensing—HYDICEurban data set, which is an image data polluted by Gaussian noise, impulse noise, stripes, atmosphere, hydrology, etc. It is an ideal data for evaluating hyperspectral denoising methods. The size of the whole image is 307×307, which contains 210 bands in total. It is easy to see that the method in the present invention and other comparison methods can remove the noise in the lightly noise-polluted band, and also preserve the local detail information of the hyperspectral image (such as figure 2 shown, where figure 2 (a) represents the original noise-containing image, (b)-(f) are images after denoising by algorithms VBM3D, LRMR, NAILRMA, WSN-LRMA, and NonLRMA respectively, and the rest of the images are operated in the same way); for moderate For noise-contaminated bands, some denoising algorithms cannot effectively remove noise. On the contrary, this method not only removes various...
example 2
[0149] Example 2: This example selects a typical dataset in the field of remote sensing—EO-1Hyperion Australia dataset. This dataset was shot on December 4, 2012. The original image size is 256×3858 and contains 242 bands in total. Due to space limitations, this method selects 200×400 sub-image blocks. This data set mostly contains vertical stripes, such as Figure 5-Figure 8 shown. It is easy to see that only this method can effectively remove the band noise and preserve the local detail information of the image.
PUM
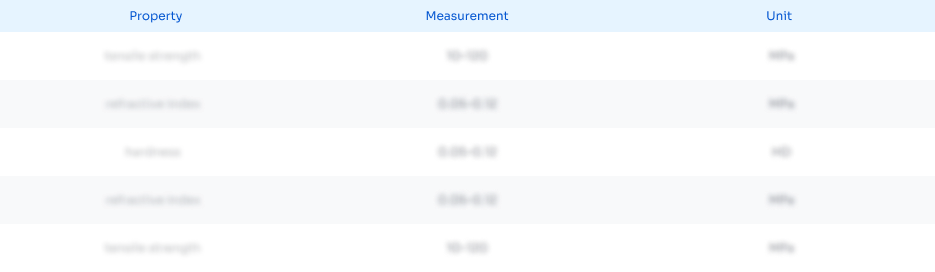
Abstract
Description
Claims
Application Information
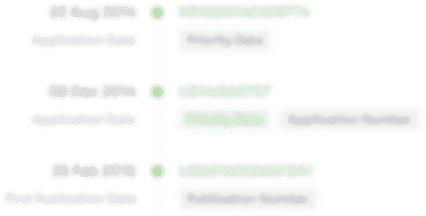
- R&D
- Intellectual Property
- Life Sciences
- Materials
- Tech Scout
- Unparalleled Data Quality
- Higher Quality Content
- 60% Fewer Hallucinations
Browse by: Latest US Patents, China's latest patents, Technical Efficacy Thesaurus, Application Domain, Technology Topic, Popular Technical Reports.
© 2025 PatSnap. All rights reserved.Legal|Privacy policy|Modern Slavery Act Transparency Statement|Sitemap|About US| Contact US: help@patsnap.com