Self-learning human body behavior recognition and anomaly detection method
An anomaly detection and self-learning technology, applied in the field of self-learning human behavior recognition and anomaly detection, which can solve the problems of high cost and inability to complete the self-learning process.
- Summary
- Abstract
- Description
- Claims
- Application Information
AI Technical Summary
Problems solved by technology
Method used
Image
Examples
Embodiment Construction
[0072] The following will clearly and completely describe the technical solutions in the embodiments of the present invention with reference to the drawings in the embodiments of the present invention.
[0073] The present invention provides a self-learning human behavior recognition and abnormal detection method, such as figure 1 As shown, the specific examples are as follows:
[0074] Step 1: Generate salient area vectors in the surveillance video, and regularly update the salient areas by self-learning;
[0075] (1) Suppose a continuous time period t i ={t 1 ,t 2 ,...t a ,t A}, where t a is a certain time point, A is the number of selected multiple discrete time points, 1≤a≤A, A≥10, statistics t i All images in the time period are combined to form an image sequence, expressed as V={v 1 ,v 2 ,...,v n ,...,v N}, N is t i The number of images in the time period, 1≤n≤N;
[0076] (2) For image v in V n The pixels in are represented as sets form: in, for v n ...
PUM
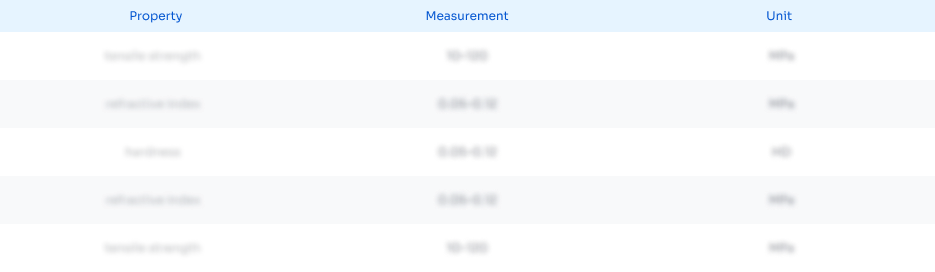
Abstract
Description
Claims
Application Information
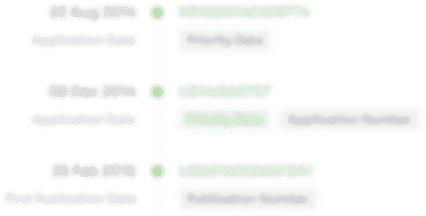
- R&D
- Intellectual Property
- Life Sciences
- Materials
- Tech Scout
- Unparalleled Data Quality
- Higher Quality Content
- 60% Fewer Hallucinations
Browse by: Latest US Patents, China's latest patents, Technical Efficacy Thesaurus, Application Domain, Technology Topic, Popular Technical Reports.
© 2025 PatSnap. All rights reserved.Legal|Privacy policy|Modern Slavery Act Transparency Statement|Sitemap|About US| Contact US: help@patsnap.com