Hyperspectral image classification method of graph convolution network based on multi-graph structure
A hyperspectral image and classification method technology, applied in the field of high-dimensional image processing, can solve the problem of unsatisfactory classification accuracy and achieve the effect of improving classification accuracy and good classification accuracy
- Summary
- Abstract
- Description
- Claims
- Application Information
AI Technical Summary
Problems solved by technology
Method used
Image
Examples
Embodiment
[0036] Such as figure 1 As shown, a hyperspectral image classification method based on a graph convolutional network with a multi-graph structure includes the following steps:
[0037] S1 reads in hyperspectral data cube H(x,y,z) and training set Among them, x and y represent the spatial pixel position, and z represents the position of the spectral band. is the set of training pixels, Y L is the corresponding training label set;
[0038] S2 takes out the hyperspectral image cube data according to the column order of the pixels, and rearranges them into a pixel data matrix V=[v 1 ,v 2 ,...,v N ] T , where N is the total number of hyperspectral image pixels, and each pixel has b features;
[0039] For example: the size of the image is originally 20*20, and the size is (400, 1) matrix using a function similar to reshape.
[0040] S3 constructs the forced nearest neighbor connection matrix and the spatial nearest neighbor connection matrix according to the pixel data mat...
PUM
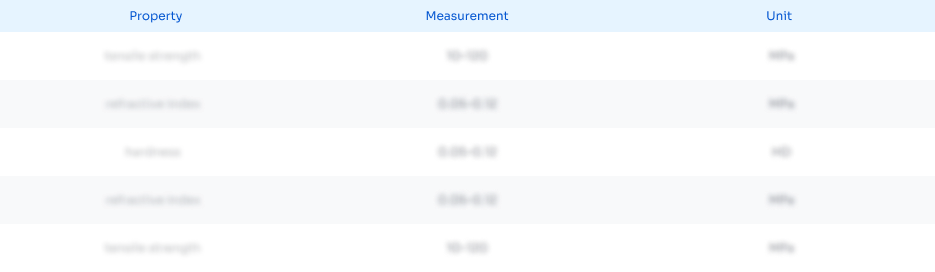
Abstract
Description
Claims
Application Information
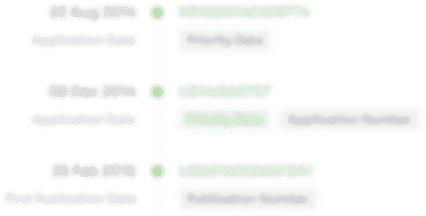
- R&D Engineer
- R&D Manager
- IP Professional
- Industry Leading Data Capabilities
- Powerful AI technology
- Patent DNA Extraction
Browse by: Latest US Patents, China's latest patents, Technical Efficacy Thesaurus, Application Domain, Technology Topic, Popular Technical Reports.
© 2024 PatSnap. All rights reserved.Legal|Privacy policy|Modern Slavery Act Transparency Statement|Sitemap|About US| Contact US: help@patsnap.com