Machine learning sample generation method for aircraft thrust fault on-line identification
A machine learning and aircraft technology, applied in instruments, general control systems, electrical testing/monitoring, etc., can solve problems such as large gaps with real data, affecting flight identification accuracy, and limited real data, so as to achieve improved identification accuracy and real data. Credibility, the effect of improving the accuracy of fault identification
- Summary
- Abstract
- Description
- Claims
- Application Information
AI Technical Summary
Problems solved by technology
Method used
Image
Examples
Embodiment Construction
[0043] The present invention will be described in detail below in conjunction with the accompanying drawings and specific embodiments.
[0044]The invention relates to a machine learning sample generation method for online identification of aircraft thrust faults, which is suitable for the field of online identification of typical power system faults during aircraft flight. Carry out data fusion generation for the flight motion information of the control system (such as flight position, speed, acceleration, attitude angle, angular velocity, etc.), and intercept the corresponding data according to the design method of the present invention as machine learning training and testing samples. The invention considers factors such as center-of-mass motion, center-of-disturbance motion, structural interference, aerodynamic force, moment, etc. of the aircraft, and introduces deviation combination cycles into the simulation model to generate data, so that the data is more authentic and r...
PUM
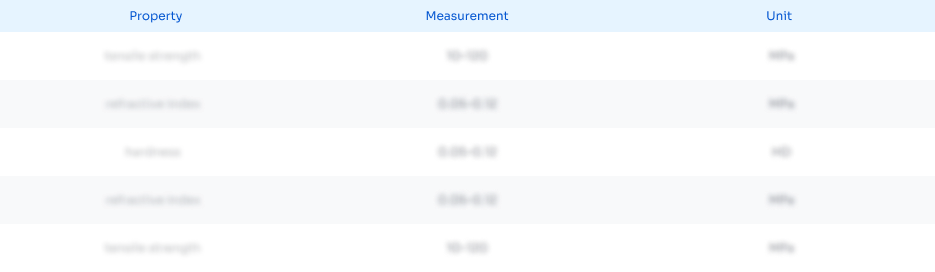
Abstract
Description
Claims
Application Information
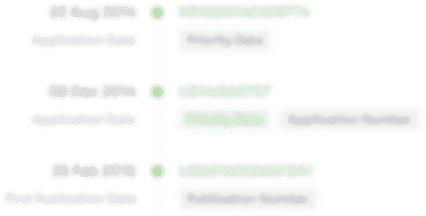
- R&D
- Intellectual Property
- Life Sciences
- Materials
- Tech Scout
- Unparalleled Data Quality
- Higher Quality Content
- 60% Fewer Hallucinations
Browse by: Latest US Patents, China's latest patents, Technical Efficacy Thesaurus, Application Domain, Technology Topic, Popular Technical Reports.
© 2025 PatSnap. All rights reserved.Legal|Privacy policy|Modern Slavery Act Transparency Statement|Sitemap|About US| Contact US: help@patsnap.com