Image generation method based on Gaussian mixture model prior variation auto-encoder
A Gaussian mixture model and autoencoder technology, applied in the field of deep learning, can solve problems such as blurry pictures, and achieve the effects of improving generation ability, strong convergence, and high training efficiency
- Summary
- Abstract
- Description
- Claims
- Application Information
AI Technical Summary
Problems solved by technology
Method used
Image
Examples
Embodiment 1
[0041] This embodiment provides an image generation method based on a Gaussian mixture model prior variational autoencoder, such as Figure 1-2 shown, including steps:
[0042] S11. Presetting generates an image training data set; wherein, the training data set is composed of several batches of training data;
[0043] S12. Build a variational autoencoder network based on the Gaussian mixture model prior;
[0044] S13. Upload the preset several batches of training data to the built variational autoencoder network, and determine the posterior distribution and prior distribution of the variational autoencoder network;
[0045] S14. Determine the relationship between the Gaussian components in the Gaussian mixture model to obtain a mapping function;
[0046] S15. Utilize the variational self-encoder network and the obtained mapping function to obtain a reconstruction loss function and a KL divergence function, and calculate the variational self-encoder network according to the o...
PUM
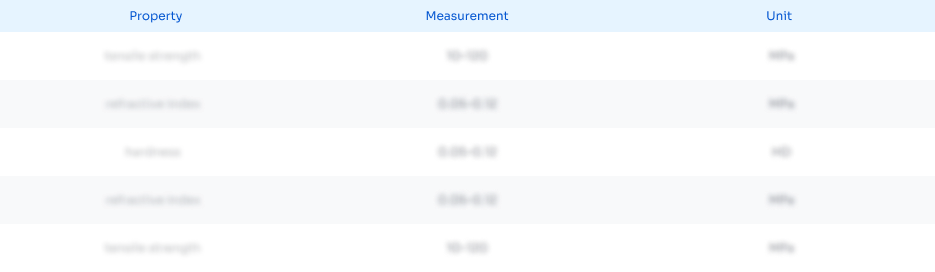
Abstract
Description
Claims
Application Information
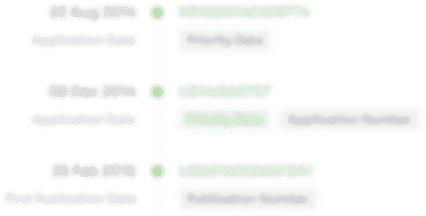
- R&D Engineer
- R&D Manager
- IP Professional
- Industry Leading Data Capabilities
- Powerful AI technology
- Patent DNA Extraction
Browse by: Latest US Patents, China's latest patents, Technical Efficacy Thesaurus, Application Domain, Technology Topic, Popular Technical Reports.
© 2024 PatSnap. All rights reserved.Legal|Privacy policy|Modern Slavery Act Transparency Statement|Sitemap|About US| Contact US: help@patsnap.com