Chaotic time sequence prediction method based on attention mechanism deep learning
A chaotic time series and deep learning technology, applied in neural learning methods, forecasting, biological neural network models, etc., can solve problems such as affecting the prediction effect of chaotic time series, distracting attention, affecting prediction accuracy and wide application.
- Summary
- Abstract
- Description
- Claims
- Application Information
AI Technical Summary
Problems solved by technology
Method used
Image
Examples
Embodiment Construction
[0042] The present invention will be further described below in conjunction with accompanying drawing.
[0043] like figure 1 As shown, a chaotic time series prediction method based on deep learning of attention mechanism includes the following steps:
[0044] Step 1. Construct a chaotic time series data set, and use the Lorenz system and Rossler system to generate chaotic time series data, which specifically includes the following sub-steps:
[0045] (a) The Lorenz system equation is described by formula (1),
[0046]
[0047] In the formula, and Indicates the derivative of the independent variable time t, a, b, c are Lorenz system parameter constants, set the initial value as a=16, b=4, c=45.92, x, y, z represent the state of the Lorenz system, set the initial value For x(0)=y(0)=z(0)=1.0, use the fourth-order Runge-Kutta method to generate a chaotic time series with Δt as the time interval;
[0048] (b) In order to make the Lorenz system completely enter the chaot...
PUM
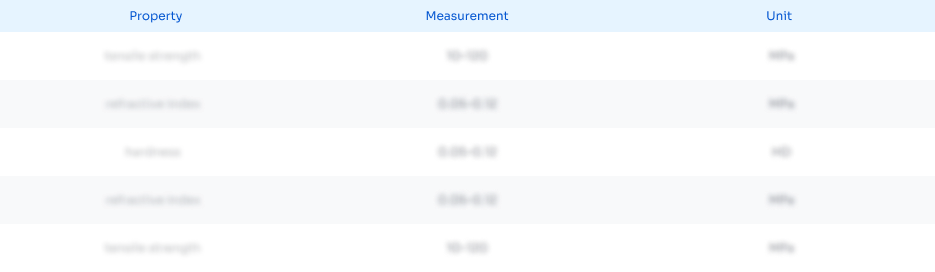
Abstract
Description
Claims
Application Information
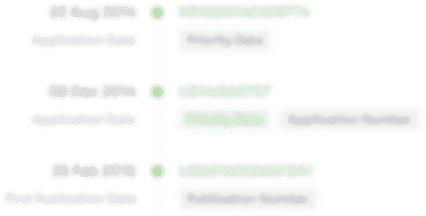
- Generate Ideas
- Intellectual Property
- Life Sciences
- Materials
- Tech Scout
- Unparalleled Data Quality
- Higher Quality Content
- 60% Fewer Hallucinations
Browse by: Latest US Patents, China's latest patents, Technical Efficacy Thesaurus, Application Domain, Technology Topic, Popular Technical Reports.
© 2025 PatSnap. All rights reserved.Legal|Privacy policy|Modern Slavery Act Transparency Statement|Sitemap|About US| Contact US: help@patsnap.com