Rate distortion optimization rapid decision-making system and method based on deep learning in HEVC intra-frame coding
A rate-distortion optimization and intra-frame coding technology, which is applied in the field of video coding, can solve the problems of HEVC encoders such as high computational complexity, unreasonableness, and reduced complexity.
- Summary
- Abstract
- Description
- Claims
- Application Information
AI Technical Summary
Problems solved by technology
Method used
Image
Examples
Embodiment Construction
[0068] Whole flow chart of the present invention is attached figure 1 Shown, the present invention is elaborated below in conjunction with accompanying drawing:
[0069] Step 1. Dataset Preparation
[0070] The present invention selects 86 video sequences as data sets from the Joint Working Group on Video Coding (JCT-VC) and Xiph.org[1]. In order to ensure the diversity of data sets, these videos have different resolution formats, including: SIF , CIF, NTSC, 4CIF, 240p, 480p, 720p, 1080p, WQXGA. A total of 86 video sequences are divided into two non-overlapping sets for training and testing, 72 of which are used to construct the training dataset and 14 are used for testing. In order to ensure the generalization performance of the network model and evaluate the model performance fairly, the videos used for training and testing are randomly selected from different resolutions. Four QP values {22, 27, 32, 37} were selected, and all sequences were encoded in full intra mode w...
PUM
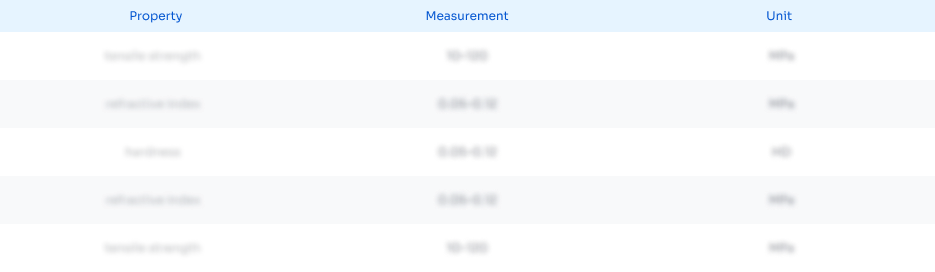
Abstract
Description
Claims
Application Information
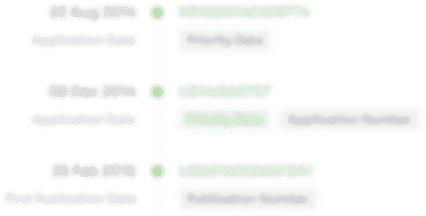
- R&D Engineer
- R&D Manager
- IP Professional
- Industry Leading Data Capabilities
- Powerful AI technology
- Patent DNA Extraction
Browse by: Latest US Patents, China's latest patents, Technical Efficacy Thesaurus, Application Domain, Technology Topic, Popular Technical Reports.
© 2024 PatSnap. All rights reserved.Legal|Privacy policy|Modern Slavery Act Transparency Statement|Sitemap|About US| Contact US: help@patsnap.com