Method of image segmentation using CN
A technology for inputting images and feature maps, applied in the field of image segmentation using CNN, which can solve problems such as large additional computing costs
- Summary
- Abstract
- Description
- Claims
- Application Information
AI Technical Summary
Problems solved by technology
Method used
Image
Examples
Embodiment Construction
[0046] A CNN is a neural network with multiple hidden layers, at least some of which are convolutional layers, where each convolutional layer is used to perform a convolution or dot product with the input provided to that layer. The CNN is implemented and operated by a computing device programmed with program codes for performing data operations according to the network structure of the CNN.
[0047] This paper discloses a CNN model that improves medical image segmentation performance relative to U-Net while maintaining or reducing computational requirements compared to U-Net. In particular, this CNN model employs a multi-scale context aggregation module to achieve the first goal of improving segmentation performance, and a CFS module to reduce computation requirements.
[0048] Before elaborating on the disclosed CNN model, an overview of U-Net is provided below. U-Net was proposed by O.RONNEBERGER, P.FISCHER and T.BROX in 2015 in "U-Net: Convolutional Networks for Biomedica...
PUM
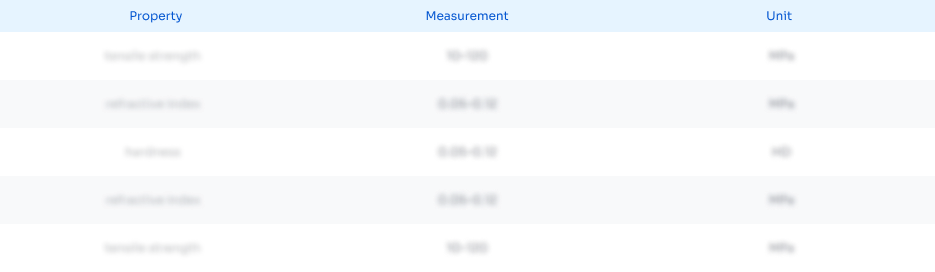
Abstract
Description
Claims
Application Information
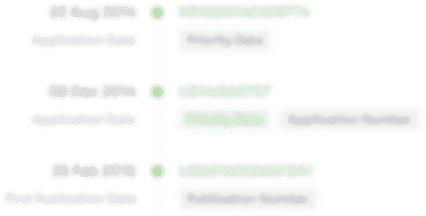
- R&D
- Intellectual Property
- Life Sciences
- Materials
- Tech Scout
- Unparalleled Data Quality
- Higher Quality Content
- 60% Fewer Hallucinations
Browse by: Latest US Patents, China's latest patents, Technical Efficacy Thesaurus, Application Domain, Technology Topic, Popular Technical Reports.
© 2025 PatSnap. All rights reserved.Legal|Privacy policy|Modern Slavery Act Transparency Statement|Sitemap|About US| Contact US: help@patsnap.com