Lightweight semantic segmentation method for high-resolution remote sensing image
A remote sensing image and semantic segmentation technology, applied in the field of remote sensing image processing, can solve the problems of low operation efficiency of segmentation algorithms and shorten the time for semantic segmentation of remote sensing images, so as to improve the accuracy of semantic segmentation, reduce the amount of parameters and calculations, and improve the operation. effect of speed
- Summary
- Abstract
- Description
- Claims
- Application Information
AI Technical Summary
Problems solved by technology
Method used
Image
Examples
Embodiment Construction
[0034] like figure 1 As shown, it is a lightweight semantic segmentation method based on high-resolution remote sensing images involved in the present invention, including the following steps:
[0035] Step A. Divide the remote sensing image sample data set into training set, verification set and test set according to the ratio of 0.5:0.15:0.35, and then make a label file for each sub-data set. The label file corresponds to the image file one by one, and stores them separately In the file directory corresponding to the hard disk, set the directory path of the read-in data before training the model, set the number of categories of the network output layer to the number of categories of the included ground objects according to the number of categories that the data set needs to classify, and set the learning rate is 0.0001, the number of iterations is 1500, the exponential decay rate is (0.9, 0.99), the regularization coefficient is 0.0002, and the loss function is the cross-ent...
PUM
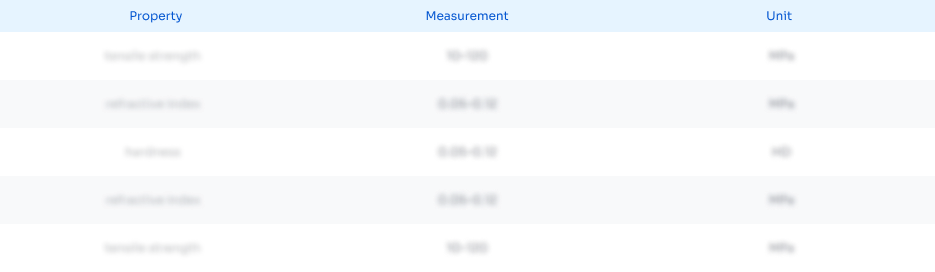
Abstract
Description
Claims
Application Information
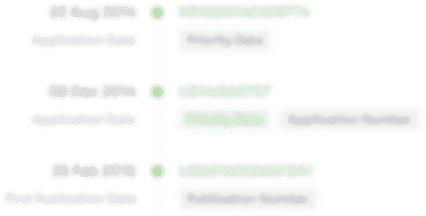
- R&D
- Intellectual Property
- Life Sciences
- Materials
- Tech Scout
- Unparalleled Data Quality
- Higher Quality Content
- 60% Fewer Hallucinations
Browse by: Latest US Patents, China's latest patents, Technical Efficacy Thesaurus, Application Domain, Technology Topic, Popular Technical Reports.
© 2025 PatSnap. All rights reserved.Legal|Privacy policy|Modern Slavery Act Transparency Statement|Sitemap|About US| Contact US: help@patsnap.com