Smooth norm limited non-negative matrix factorization clustering method based on graph regularization
A technology of non-negative matrix decomposition and clustering method, which is applied in the field of view clustering, can solve problems such as poor clustering effect, and achieve the effect of enhancing identification ability, improving clustering performance, and strong identification ability
- Summary
- Abstract
- Description
- Claims
- Application Information
AI Technical Summary
Problems solved by technology
Method used
Image
Examples
Embodiment Construction
[0014] In order to more clearly illustrate the embodiments of the present invention or the technical solutions in the prior art, the specific implementation manners of the present invention will be described below with reference to the accompanying drawings. Obviously, the accompanying drawings in the following description are only some embodiments of the present invention, and those skilled in the art can obtain other accompanying drawings based on these drawings and obtain other implementations.
[0015] like figure 1 Shown is a schematic flow chart of the clustering method based on graph regularization-based smooth norm constrained non-negative matrix factorization (GSCNMF) provided by the present invention, including:
[0016] S10 obtains the view to be clustered and constructs the nearest neighbor graph;
[0017] S20 builds L based on graph regularization P The objective function of the smooth norm restricted non-negative matrix factorization, which includes a smooth r...
PUM
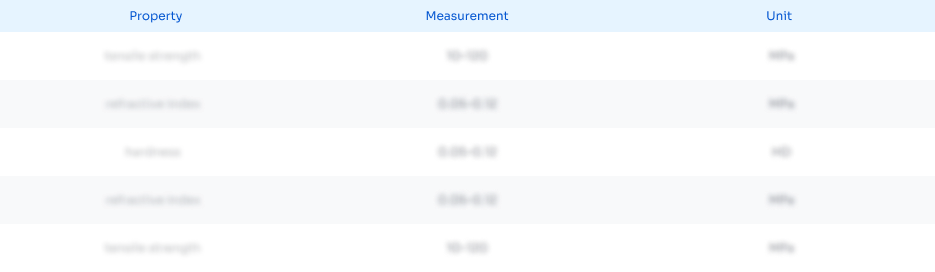
Abstract
Description
Claims
Application Information
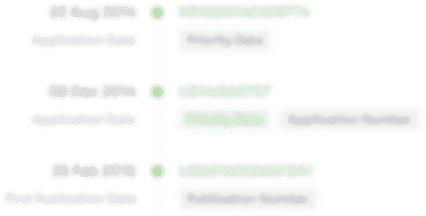
- R&D Engineer
- R&D Manager
- IP Professional
- Industry Leading Data Capabilities
- Powerful AI technology
- Patent DNA Extraction
Browse by: Latest US Patents, China's latest patents, Technical Efficacy Thesaurus, Application Domain, Technology Topic.
© 2024 PatSnap. All rights reserved.Legal|Privacy policy|Modern Slavery Act Transparency Statement|Sitemap