Nuclear magnetic resonance image classification method based on multi-strategy batch type active learning
An MRI, active learning technology, applied in neural learning methods, character and pattern recognition, recognizing medical/anatomical patterns, etc., can solve problems such as redundant information of samples, achieve high classification accuracy, and improve classification accuracy. , the effect of efficient diagnosis
- Summary
- Abstract
- Description
- Claims
- Application Information
AI Technical Summary
Problems solved by technology
Method used
Image
Examples
Embodiment 1
[0074] Embodiment 1, a kind of nuclear magnetic resonance image classification method based on multi-strategy batch type active learning, comprises the following steps:
[0075] S1: Obtain the brain MRI images of three types of subjects with normal cognition, mild cognitive impairment and Alzheimer's disease as the original data set. The data set selects the data of 571 subjects, among which Alzheimer's disease There were 192 subjects, 171 subjects with mild cognitive impairment, and 208 subjects with normal cognition. The original data set was preprocessed to obtain an unlabeled sample set, an unlabeled validation set, and an unlabeled test set;
[0076] Wherein: preprocessing the original data set includes the following steps:
[0077] S1-1: Use SPM12 to preprocess the acquired nuclear magnetic resonance image, perform head correction, registration and segmentation operations on the NIFTI format nuclear magnetic resonance image, and obtain three images of gray matter, white...
PUM
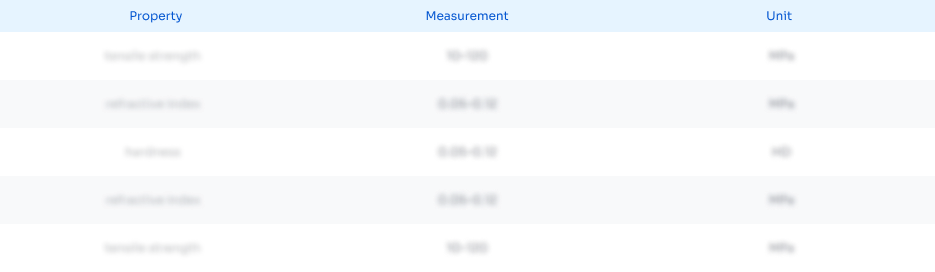
Abstract
Description
Claims
Application Information
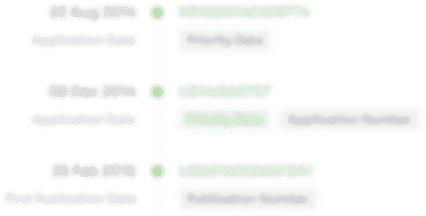
- R&D
- Intellectual Property
- Life Sciences
- Materials
- Tech Scout
- Unparalleled Data Quality
- Higher Quality Content
- 60% Fewer Hallucinations
Browse by: Latest US Patents, China's latest patents, Technical Efficacy Thesaurus, Application Domain, Technology Topic, Popular Technical Reports.
© 2025 PatSnap. All rights reserved.Legal|Privacy policy|Modern Slavery Act Transparency Statement|Sitemap|About US| Contact US: help@patsnap.com