Virtual network function deployment optimization algorithm based on deep reinforcement learning
A virtual network function and reinforcement learning technology, applied in the field of virtual network function deployment optimization algorithms, can solve problems such as increased queue overflow probability, data backlog, and no consideration of dynamic changes in the environment, and achieve the effect of improving resource utilization.
- Summary
- Abstract
- Description
- Claims
- Application Information
AI Technical Summary
Problems solved by technology
Method used
Image
Examples
Embodiment Construction
[0038] The specific embodiments of the present invention will be described in detail below with reference to the accompanying drawings.
[0039] See figure 1 , figure 1 It is a schematic diagram of a scenario based on the NFV / SDN architecture of the present invention.
[0040] Abstract the physical network as an undirected graph G P =(V P , E P ), V P Represents the physical node, that is, the general physical server, which provides the VNF with instantiated CPU resources, and each underlying general physical server can instantiate multiple VNFs, E P Represents a collection of physical links. Each underlying general server v∈V P The CPU capacity is Physical link e connecting adjacent general servers v and u vu The bandwidth capacity is And the transmission delay is τ uv . Due to the low CPU resource utilization of some general-purpose servers, this article sets a CPU resource threshold for general-purpose servers That is, the CPU resources of the general server in each time sl...
PUM
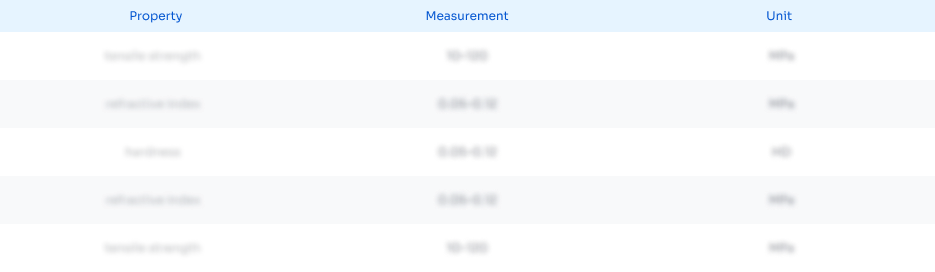
Abstract
Description
Claims
Application Information
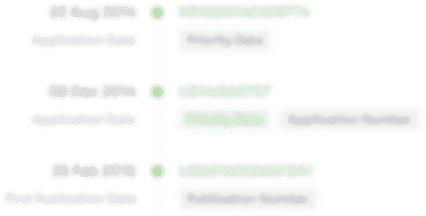
- R&D
- Intellectual Property
- Life Sciences
- Materials
- Tech Scout
- Unparalleled Data Quality
- Higher Quality Content
- 60% Fewer Hallucinations
Browse by: Latest US Patents, China's latest patents, Technical Efficacy Thesaurus, Application Domain, Technology Topic, Popular Technical Reports.
© 2025 PatSnap. All rights reserved.Legal|Privacy policy|Modern Slavery Act Transparency Statement|Sitemap|About US| Contact US: help@patsnap.com