Image anti-theft system and method based on deep learning
A deep learning and anti-theft system technology, applied in the field of machine learning, can solve problems such as affecting the display of image content, weak security, and impact on display effects, and achieve the effect of solving hardware computing power problems and preventing edge problems.
- Summary
- Abstract
- Description
- Claims
- Application Information
AI Technical Summary
Problems solved by technology
Method used
Image
Examples
Embodiment 1
[0035] This embodiment discloses an image anti-theft system based on deep learning, including a generator, a discriminator, and an optimizer. The optimizer configures a loss function; the generator and the discriminator form an adversarial network. The generator is responsible for the generation of the intermediate image and the final image, and the discriminator is responsible for distinguishing the true and false of the final image. The two are trained alternately to improve the consistency between the output image of the generator and the input image.
[0036] The generator is a neural network structure. Considering that when the resolution of the input image is odd, the convolution or pooling operation will cause the image to fail to return to the original resolution if strides>1, so the entire generator is not down-sampled. In order to reduce the model parameters, a cyclic residual block is constructed, the residual is the method of cancatenate (the number of cycles is 2)...
Embodiment 2
[0048] Such as Figure 4 As shown, this embodiment uses the system in Embodiment 1 to Figure 4 (a) As the original image, it is input into the generator, and the obtained intermediate image is as follows Figure 4 As shown in (b), save it to the server for user access. On this basis, configure the penultimate layer and the last layer of the generator into the display unit of the system. When an anonymous user accesses the intermediate image, the intermediate image is used as the input of the penultimate layer, and finally generated by the output of the last layer. The image is displayed to the user, as Figure 4 The generated image of (c). Also, the images displayed by the display unit cannot be downloaded.
Embodiment 3
[0050] This embodiment discloses an image anti-theft system based on deep learning, including a generator, a discriminator, and an optimizer. The optimizer configures a loss function; the generator and the discriminator form an adversarial network. The generator generates the input original image into an intermediate image and the final generated image, and the generated image is consistent with the original image. The discriminator is responsible for judging whether the final image is true or false, that is, judging whether the generated image is consistent with the original image; the two are alternately trained to improve The consistency between the output image of the generator (ie, the generated image) and the input image (ie, the original image).
[0051] Taking 64*64 resolution as the size of image training as an example, the network structure of the generator is as follows figure 2 As shown, (a) and (b) are connected to obtain the network structure of the generator. ...
PUM
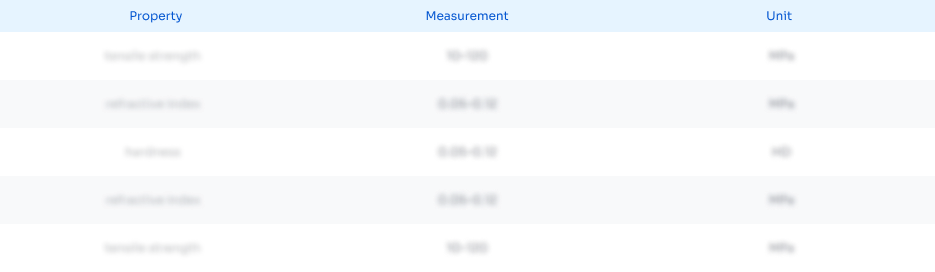
Abstract
Description
Claims
Application Information
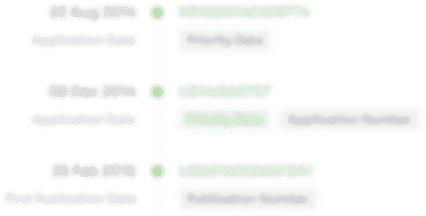
- R&D Engineer
- R&D Manager
- IP Professional
- Industry Leading Data Capabilities
- Powerful AI technology
- Patent DNA Extraction
Browse by: Latest US Patents, China's latest patents, Technical Efficacy Thesaurus, Application Domain, Technology Topic, Popular Technical Reports.
© 2024 PatSnap. All rights reserved.Legal|Privacy policy|Modern Slavery Act Transparency Statement|Sitemap|About US| Contact US: help@patsnap.com