Collaborative filtering recommendation method based on parallel auto-encoder
A collaborative filtering recommendation and self-encoding technology, applied in neural learning methods, marketing, biological neural network models, etc., can solve the problems of performance degradation of personalized recommendation, difficulty in capturing different features, and difficulty in obtaining auxiliary information, achieving strong robustness. Robustness and practicality, the effect of reducing time complexity, reducing instability
- Summary
- Abstract
- Description
- Claims
- Application Information
AI Technical Summary
Problems solved by technology
Method used
Image
Examples
Embodiment Construction
[0043] Such asfigure 1 A collaborative filtering recommendation method based on a parallel autoencoder is shown, including the following steps:
[0044] Step 1. Build a sparse autoencoder model to complete the objective function of user latent feature representation, learn high-level abstract features based on users, and obtain the reconstruction matrix of the user rating matrix;
[0045] Step 2. Build a graph-regularized autoencoder model to complete the objective function of latent feature representation of the product, learn high-level abstract features based on the product, and obtain the reconstruction matrix of the product rating matrix;
[0046] Step 3: Multiply the reconstruction matrix based on the user rating matrix and the reconstruction matrix based on the product rating matrix to obtain the prediction matrix that the user is interested in the product, and recommend the user based on the result.
[0047] The method is specifically carried out as follows:
[0048] ...
PUM
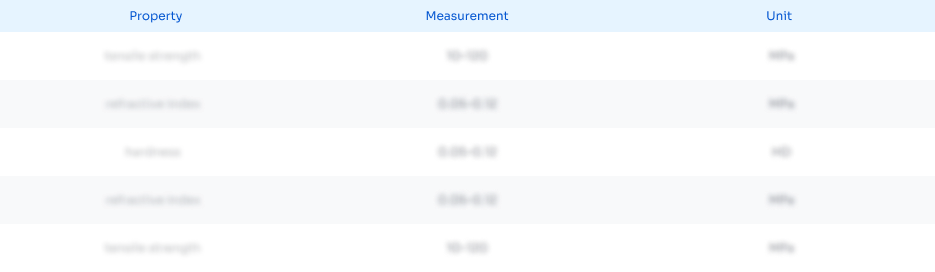
Abstract
Description
Claims
Application Information
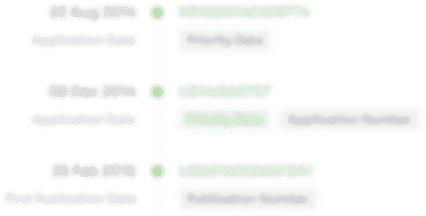
- R&D
- Intellectual Property
- Life Sciences
- Materials
- Tech Scout
- Unparalleled Data Quality
- Higher Quality Content
- 60% Fewer Hallucinations
Browse by: Latest US Patents, China's latest patents, Technical Efficacy Thesaurus, Application Domain, Technology Topic, Popular Technical Reports.
© 2025 PatSnap. All rights reserved.Legal|Privacy policy|Modern Slavery Act Transparency Statement|Sitemap|About US| Contact US: help@patsnap.com