Semantic segmentation method based on reverse attention model
An attention model and semantic segmentation technology, applied in the field of image processing, can solve complex problems, increase the number of model learning parameters, etc., to achieve the effect of speeding up
- Summary
- Abstract
- Description
- Claims
- Application Information
AI Technical Summary
Problems solved by technology
Method used
Image
Examples
Embodiment Construction
[0038] In order to elaborate on the purpose, technical solutions and advantages of the present invention, the present invention will be further described in detail below in conjunction with specific implementation steps and accompanying drawings.
[0039] The present invention provides a semantic segmentation method based on the reverse attention model. In the commonly used full convolutional network (CNN) semantic segmentation model, the reverse attention model is introduced, and the attention view of the high-level output features of the model is Reverse the low-level features of the model and perform multi-feature fusion to maintain the boundary information in the segmentation results, while filtering part of the noise information to improve the accuracy of the semantic segmentation results.
[0040] Since the present invention adds a loss function based on Gumbe softmax to the output features of the last layer of the basic semantic segmentation model after attention self-en...
PUM
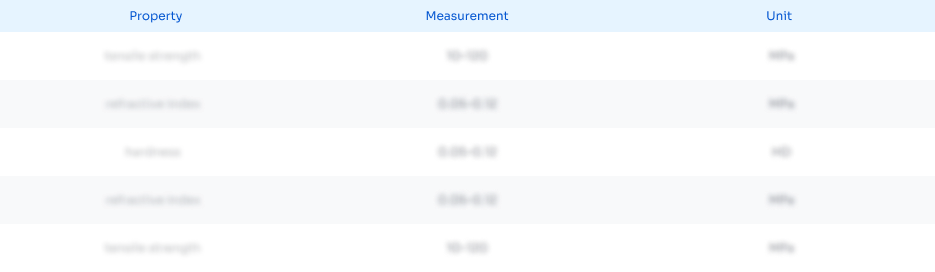
Abstract
Description
Claims
Application Information
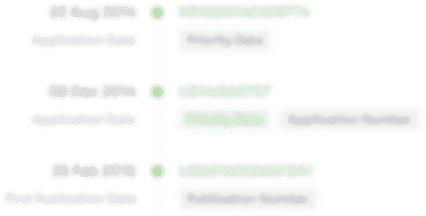
- R&D Engineer
- R&D Manager
- IP Professional
- Industry Leading Data Capabilities
- Powerful AI technology
- Patent DNA Extraction
Browse by: Latest US Patents, China's latest patents, Technical Efficacy Thesaurus, Application Domain, Technology Topic.
© 2024 PatSnap. All rights reserved.Legal|Privacy policy|Modern Slavery Act Transparency Statement|Sitemap