Semi-supervised polarimetric SAR ground object classification method based on characteristic mixup
A ground object classification and semi-supervised technology, applied in the field of image processing, can solve the problems of long training time, high time complexity, and low efficiency, and achieve the effects of enhancing robustness, improving classification accuracy, and improving performance
- Summary
- Abstract
- Description
- Claims
- Application Information
AI Technical Summary
Problems solved by technology
Method used
Image
Examples
Embodiment Construction
[0055] The present invention provides a semi-supervised polarization SAR object classification method based on feature mixup, which includes preparing a data set; making a data set; data preprocessing; designing a network structure; extracting primary features from a double-branch network; The branch predicts the object category; trains the object classification network; inputs the test sample into the network to predict the object category; evaluates the network performance. The present invention effectively overcomes the problem of redundant pixels affecting classification results caused by directly mixing up the input in the prior art, the problem of using the mean square error to calculate the consistency loss will enhance the memory capacity of the network, and the problem of unreliable single-branch prediction. It greatly improves the performance of the network and enhances the robustness of the network. The invention can be used for land cover type discrimination, and c...
PUM
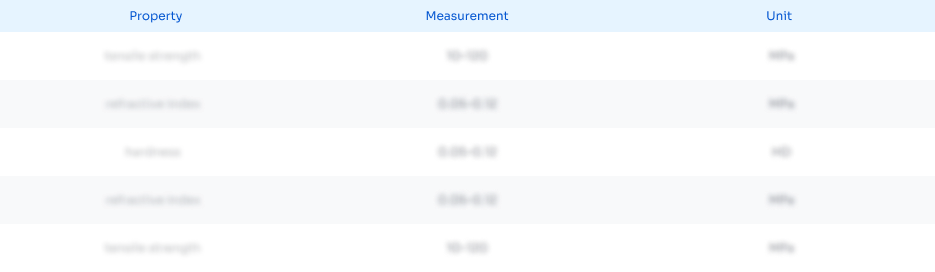
Abstract
Description
Claims
Application Information
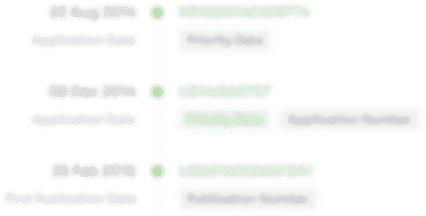
- R&D Engineer
- R&D Manager
- IP Professional
- Industry Leading Data Capabilities
- Powerful AI technology
- Patent DNA Extraction
Browse by: Latest US Patents, China's latest patents, Technical Efficacy Thesaurus, Application Domain, Technology Topic, Popular Technical Reports.
© 2024 PatSnap. All rights reserved.Legal|Privacy policy|Modern Slavery Act Transparency Statement|Sitemap|About US| Contact US: help@patsnap.com