Foggy day vehicle detection method based on deep learning
A vehicle detection and deep learning technology, applied in the field of vehicle detection in foggy weather based on deep learning, can solve problems such as poor detection results and redundant, and achieve the effects of improving efficiency, simplifying network structure, and improving target detection accuracy.
- Summary
- Abstract
- Description
- Claims
- Application Information
AI Technical Summary
Problems solved by technology
Method used
Image
Examples
Embodiment Construction
[0052] The present invention is described in further detail below in conjunction with accompanying drawing:
[0053] Such as figure 1 As shown, a vehicle detection method in foggy weather based on deep learning includes the following steps:
[0054] Step 1), collecting traffic vehicle pictures in foggy weather;
[0055] In the collected images of traffic vehicles in foggy weather, information other than the vehicle is used as the background. The specific collected images of traffic vehicles in foggy weather are as follows: figure 2 shown.
[0056] Step 2), image preprocessing is carried out to the collected foggy traffic vehicle picture, specifically image defogging, inversion and symmetry processing are carried out to the foggy traffic vehicle picture, the data set is expanded, and the robustness of network training is increased;
[0057] Specifically, the dark channel prior defogging method is used to preprocess the image, and the physical model based on the dark channel...
PUM
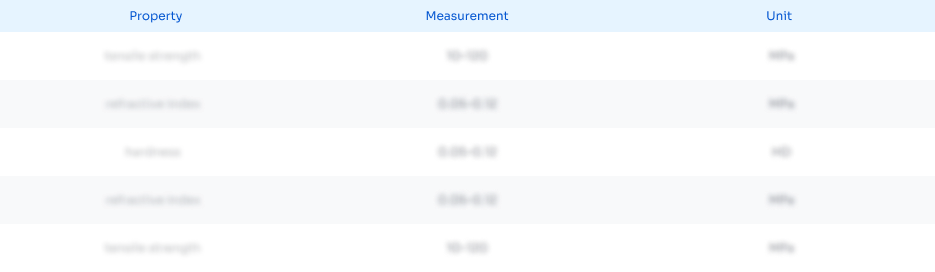
Abstract
Description
Claims
Application Information
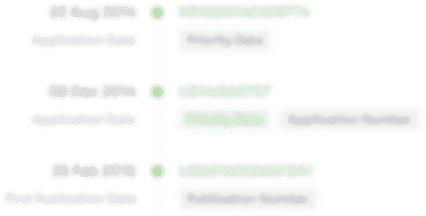
- R&D Engineer
- R&D Manager
- IP Professional
- Industry Leading Data Capabilities
- Powerful AI technology
- Patent DNA Extraction
Browse by: Latest US Patents, China's latest patents, Technical Efficacy Thesaurus, Application Domain, Technology Topic, Popular Technical Reports.
© 2024 PatSnap. All rights reserved.Legal|Privacy policy|Modern Slavery Act Transparency Statement|Sitemap|About US| Contact US: help@patsnap.com