Remote sensing image water area automatic extraction method and system based on deep learning
A remote sensing image and deep learning technology, applied in the field of geographic information, can solve the problem of time-consuming and labor-intensive methods of water extraction from remote sensing images
- Summary
- Abstract
- Description
- Claims
- Application Information
AI Technical Summary
Problems solved by technology
Method used
Image
Examples
specific Embodiment approach 1
[0014] Specific implementation mode 1. Combination figure 1 Describe this embodiment, the deep learning-based remote sensing image waters automatic extraction method described in this embodiment, it comprises the following steps:
[0015] Step (1), download the Sentinel-2 data (S2A MSIL1C) of the European Space Agency, open the CMD console, perform atmospheric correction through the command L2A_Process in Sen2cor, and resample the corrected data through the SNAP software (raster->geometric operations->resampling) to obtain the data of each band of remote sensing images that can be processed by ENVI 5.3 software.
[0016] Step (2), the normalized difference water index NDWI (NDWI=(Green-NIR) / (Green+NIR)), the improved water index model NDWI3 (NDWI3= (NIR-SWIR-2) / (NIR+SWIR-2)), improved normalized difference water index MNDWI (MNDWI=(Green-SWIR-1) / (Green+SWIR-1)), enhanced water index EWI (EWI=(Green-NIR-SWIR-1) / (Green+NIR+SWIR-1)), Normalized Difference Vegetation Cover Index...
specific Embodiment approach 2
[0023] Specific embodiment two, combine figure 2 , image 3 , Figure 4 and Figure 5 Describe this embodiment, the remote sensing image water area segmentation model WE-Net described in this embodiment, it comprises the following steps:
[0024] Encoding step: In the encoding stage, the feature information of the water area is extracted through convolution and pooling. In the encoding stage, 12 grayscale images are used as input data, and the feature map is obtained by convolving and fusing the input data. Every time the feature map passes through a pooling layer, it is a scale. There are 5 scales including the scale of the original image, namely 256*256*32 , 128*128*64, 64*64*128, 32*32*256, and 16*16*512; after the pooling layer, the size of the feature map is halved and the number of channels is doubled, and then two convolutional neural networks The network extracts the water feature information of the image.
[0025] Decoding step: In the decoding stage, the image ...
PUM
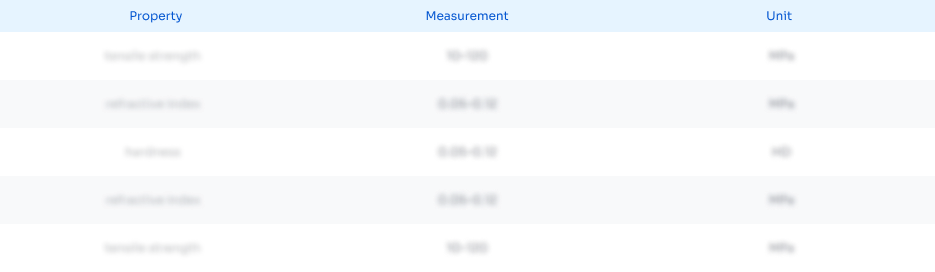
Abstract
Description
Claims
Application Information
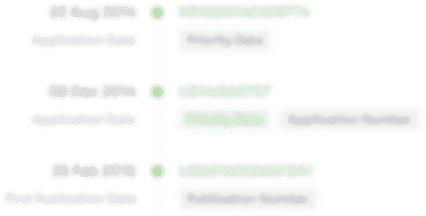
- R&D Engineer
- R&D Manager
- IP Professional
- Industry Leading Data Capabilities
- Powerful AI technology
- Patent DNA Extraction
Browse by: Latest US Patents, China's latest patents, Technical Efficacy Thesaurus, Application Domain, Technology Topic.
© 2024 PatSnap. All rights reserved.Legal|Privacy policy|Modern Slavery Act Transparency Statement|Sitemap