Wide-azimuth pre-stack seismic reflection mode analysis method of tensor depth self-encoding network
A self-encoding network and pre-stack seismic technology, applied in the field of wide-azimuth pre-stack seismic reflection mode analysis, can solve problems that are not suitable for seismic data reflection mode analysis
- Summary
- Abstract
- Description
- Claims
- Application Information
AI Technical Summary
Problems solved by technology
Method used
Image
Examples
Embodiment Construction
[0055] The technical principle of the present invention will be described below.
[0056] This invention will use the following notation: Tensors are represented using bold calligraphic letters, such as Matrices are represented by bold uppercase letters, such as T; vectors are represented by bold lowercase letters, such as t; scalars are represented by lowercase letters, such as t. Tensor space for 3D seismic data Use i, j, k to denote the xline, inline and time dimensions of 3D seismic data. Therefore, i∈[N x ], j ∈ [N y ], k∈[N t ], where [N] represents the set {1, 2, ..., N}. for tensor elements in available express. The i-th horizontal slice (horizontal slice), the j-th side slice (lateral slice) and the k-th frontal slice (frontal slice) are denoted as where the k-th frontal slice is available express.
[0057] Assume is a three-dimensional tensor of dimension l×m×n. Fixed third dimension, forward slicing It is a matrix with a dimension of l×m, where...
PUM
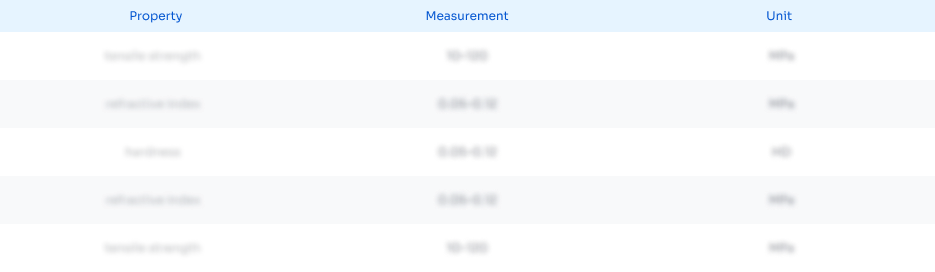
Abstract
Description
Claims
Application Information
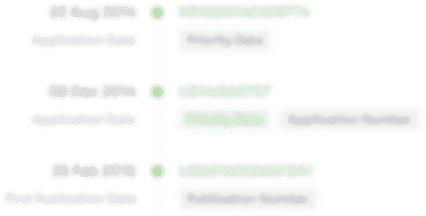
- R&D
- Intellectual Property
- Life Sciences
- Materials
- Tech Scout
- Unparalleled Data Quality
- Higher Quality Content
- 60% Fewer Hallucinations
Browse by: Latest US Patents, China's latest patents, Technical Efficacy Thesaurus, Application Domain, Technology Topic, Popular Technical Reports.
© 2025 PatSnap. All rights reserved.Legal|Privacy policy|Modern Slavery Act Transparency Statement|Sitemap|About US| Contact US: help@patsnap.com