Complex equipment fault prediction method and system based on LPP-HMM method
A LPP-HMM, fault prediction technology, applied in prediction, neural learning methods, data processing applications, etc., can solve the problem that the signal processing method is difficult to effectively obtain fault characteristics, cannot identify intermittent faults and random faults, and is difficult to fully reflect the system state, etc. problems, to achieve the effect of improving the fault identification rate, facilitating fault identification, and achieving excellent results
- Summary
- Abstract
- Description
- Claims
- Application Information
AI Technical Summary
Problems solved by technology
Method used
Image
Examples
Embodiment 1
[0031] A complex equipment fault prediction method and system based on the LPP-HMM method, including the following steps;
[0032] S1. Data processing and feature extraction: collect sample data, and use the LPP algorithm to preprocess the sample data;
[0033] S2. Determine the hidden state number L of the HMM model according to the actual situation of the equipment, set the initial parameters of the model, establish the initial HMM model λ=(π,A,B), and perform maximum likelihood estimation for each state type , select any k eigenvectors to form a set of state sequences to train its HMM model until the convergence condition is met, the state type is a hidden state, and each state type needs to train an HMM model;
[0034] S3. Input the original feature information in the preprocessed data in S1 into the fuzzy neural network, and perform network calculation to select n HMM models with the strongest correlation;
[0035] S4. According to the state prediction information of the...
Embodiment 2
[0052] The present invention introduces nonlinear manifold learning into complex equipment fault diagnosis, and proposes a fault diagnosis method using LPP and HMM, which directly learns original high-dimensional fault data and converts complex high-dimensional space into low-dimensional features space to achieve dimensionality reduction and extract the inherent low-dimensional manifold features of the data. Through simulation experiments, it is shown that this method can better preserve the overall structure information in the fault data, which is beneficial to fault identification. The LPP algorithm can be used to reduce the nonlinear fault characteristics of analog circuits, and the effect is better than that of linear dimensionality reduction methods such as PCA. The hybrid HMM classifier reflects the real state of each stage of the system. A good recognition effect can effectively identify the early faults and intermediate states of the system. Combining the LPP method wi...
PUM
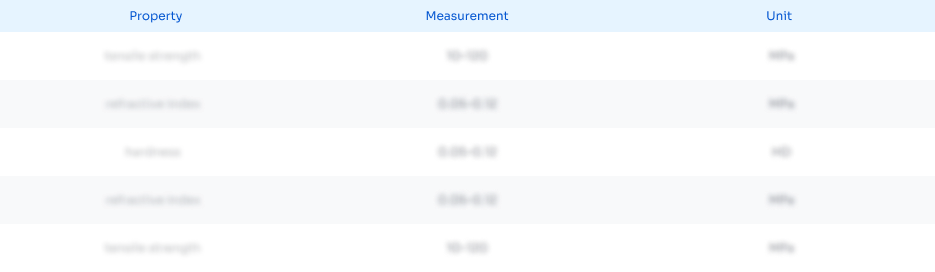
Abstract
Description
Claims
Application Information
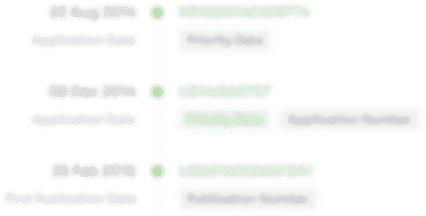
- R&D Engineer
- R&D Manager
- IP Professional
- Industry Leading Data Capabilities
- Powerful AI technology
- Patent DNA Extraction
Browse by: Latest US Patents, China's latest patents, Technical Efficacy Thesaurus, Application Domain, Technology Topic, Popular Technical Reports.
© 2024 PatSnap. All rights reserved.Legal|Privacy policy|Modern Slavery Act Transparency Statement|Sitemap|About US| Contact US: help@patsnap.com