Optical remote sensing image forest land classification method based on cascaded deep convolutional neural network
A deep convolution and neural network technology, applied in the field of remote sensing, can solve the problems of less utilization, difficulty in manual labeling, difficult sample data, etc., and achieve the effect of saving labor costs and improving investigation efficiency
- Summary
- Abstract
- Description
- Claims
- Application Information
AI Technical Summary
Problems solved by technology
Method used
Image
Examples
Embodiment Construction
[0028] The present invention comprises the following steps,
[0029] A. Using the image semantic segmentation deep convolutional network to realize the classification of large-grained ground objects in the image, and to realize the division of forest land and non-wood land areas;
[0030] B. Using a deep convolutional neural network for image classification to achieve fine-grained and fine-grained division of woodland areas.
[0031] In step A, the DeepLab-V3 deep network is first trained offline, and then the online image segmentation is based on the DeepLab-V3 deep network.
[0032] The offline training of the DeepLab-V3 deep network includes the following steps,
[0033] Firstly, multiple small-scale remote sensing images are cropped from the large-scale remote sensing images, and then these small-scale remote sensing images are labeled; next, the small-scale remote sensing images and the real value labeled images are expanded; finally, the DeepLab-V3 network is input into...
PUM
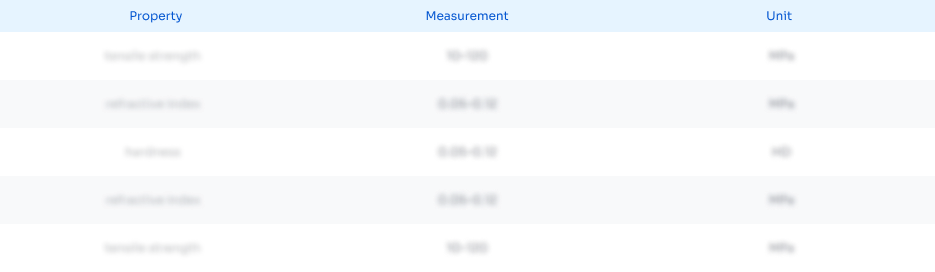
Abstract
Description
Claims
Application Information
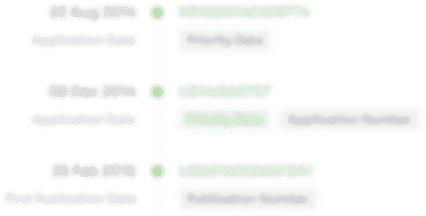
- Generate Ideas
- Intellectual Property
- Life Sciences
- Materials
- Tech Scout
- Unparalleled Data Quality
- Higher Quality Content
- 60% Fewer Hallucinations
Browse by: Latest US Patents, China's latest patents, Technical Efficacy Thesaurus, Application Domain, Technology Topic, Popular Technical Reports.
© 2025 PatSnap. All rights reserved.Legal|Privacy policy|Modern Slavery Act Transparency Statement|Sitemap|About US| Contact US: help@patsnap.com