Training method of deep convolutional neural network for face recognition
A deep convolution and face recognition technology, applied in the training field of deep convolutional neural network, can solve problems such as difficult convergence, single action type, and difficult aggregation of feature points, so as to speed up convergence, improve degrees of freedom, and class-to-class more diffuse effects
- Summary
- Abstract
- Description
- Claims
- Application Information
AI Technical Summary
Problems solved by technology
Method used
Image
Examples
Embodiment Construction
[0046] The present invention will be further described below in conjunction with specific examples.
[0047] Such as figure 1 As shown, the training method of the deep convolutional neural network for face recognition provided by this example includes the following steps:
[0048] 1) Prepare the face image data set, including training set and verification set. Before training or verification, several preprocessing steps are required for the face image data in the dataset, including face detection and alignment, image pixel value normalization, image size normalization, image enhancement, and dataset cleaning, etc. In this example, the image pixel value is normalized to a 32-bit floating-point number between 0 and 1, and the image size is normalized to 112×112×3 (respectively representing the length, width and color channel number of the input image). The used Image enhancement methods include gray scale linear transformation, histogram equalization transformation, pixel colo...
PUM
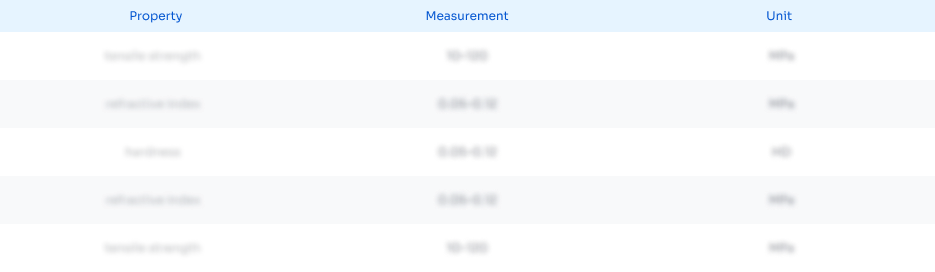
Abstract
Description
Claims
Application Information
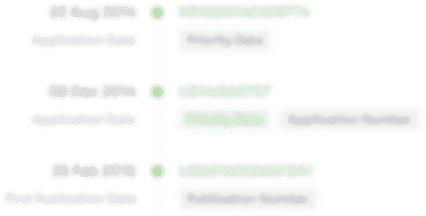
- R&D Engineer
- R&D Manager
- IP Professional
- Industry Leading Data Capabilities
- Powerful AI technology
- Patent DNA Extraction
Browse by: Latest US Patents, China's latest patents, Technical Efficacy Thesaurus, Application Domain, Technology Topic, Popular Technical Reports.
© 2024 PatSnap. All rights reserved.Legal|Privacy policy|Modern Slavery Act Transparency Statement|Sitemap|About US| Contact US: help@patsnap.com