Medical image small lesion segmentation method
A technology of medical imaging and lesions, applied in the field of semantic segmentation of computer vision, can solve the problem of insufficient segmentation accuracy of 3D small objects, and achieve the effect of high precision
- Summary
- Abstract
- Description
- Claims
- Application Information
AI Technical Summary
Problems solved by technology
Method used
Image
Examples
Embodiment Construction
[0037] combined with figure 1 The technical scheme of the present invention will be further described.
[0038] like figure 1As shown, the present invention provides a method for segmenting small lesions in medical images, including a segmentation network composed of a first stage of rough segmentation, a second stage of refinement, and an attention module for segmenting error areas. The segmentation network implements small medical images in the following steps: Lesion segmentation:
[0039] S1, the first-stage network trains the sampled and processed original image in a 5-fold cross-validation manner to obtain the segmentation result of each training data; wherein, the first-stage training adopts a 5-fold cross-validation method to obtain the predicted segmentation As a result, the steps are as follows:
[0040] 1) The image is down-sampled to be input to the network for training, and the method used for down-sampling is maxpooling. Pooling is a nonlinear operation tha...
PUM
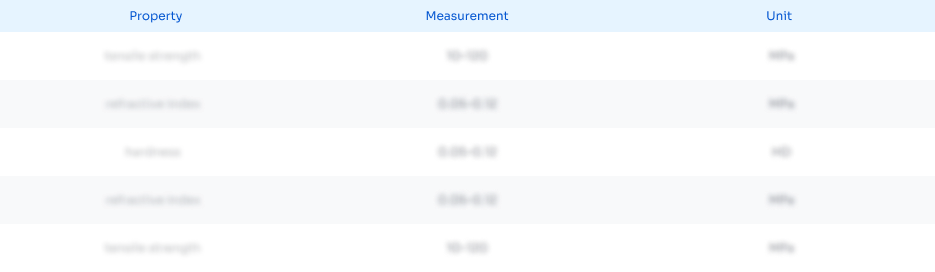
Abstract
Description
Claims
Application Information
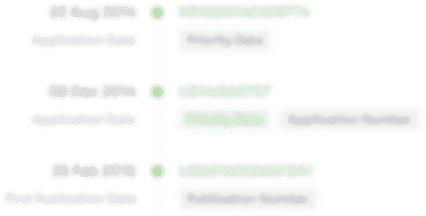
- R&D Engineer
- R&D Manager
- IP Professional
- Industry Leading Data Capabilities
- Powerful AI technology
- Patent DNA Extraction
Browse by: Latest US Patents, China's latest patents, Technical Efficacy Thesaurus, Application Domain, Technology Topic, Popular Technical Reports.
© 2024 PatSnap. All rights reserved.Legal|Privacy policy|Modern Slavery Act Transparency Statement|Sitemap|About US| Contact US: help@patsnap.com