Traffic flow prediction method based on global diffusion convolution residual network
A forecasting method, traffic forecasting technology, applied in traffic flow detection, road vehicle traffic control system, forecasting, etc., can solve the problem of failing to simultaneously capture the global and local temporal and spatial correlations of the traffic network, and achieve good forecasting performance
- Summary
- Abstract
- Description
- Claims
- Application Information
AI Technical Summary
Problems solved by technology
Method used
Image
Examples
Embodiment Construction
[0036] Figure 1-8 It is the best embodiment of the present invention, below in conjunction with the attached Figure 1-8 The present invention will be further described.
[0037] Such as figure 1 As shown, a traffic flow prediction method based on the global diffusion convolution residual network (hereinafter referred to as the traffic flow prediction method), includes the following steps:
[0038] Step 1. Establish a traffic flow prediction model based on the global diffusion convolutional residual network.
[0039] In this traffic flow forecasting method, a global diffusion convolution residual network (GDCRN for short) is used, and a traffic flow forecasting model based on the global diffusion convolution residual network is established, and in this traffic flow forecasting method, a The traffic flow prediction model based on the Global Diffusion Convolutional Residual Network is referred to as "GDCRN model" for short. At the same time, in this traffic flow prediction ...
PUM
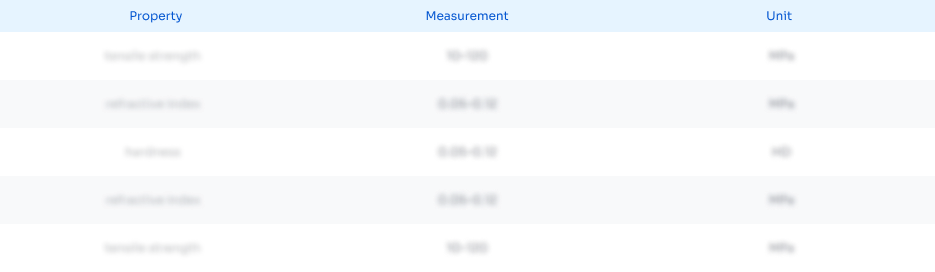
Abstract
Description
Claims
Application Information
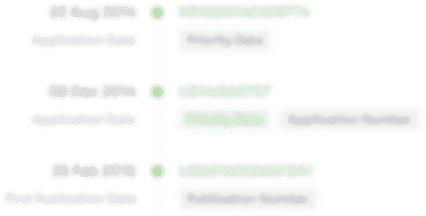
- R&D Engineer
- R&D Manager
- IP Professional
- Industry Leading Data Capabilities
- Powerful AI technology
- Patent DNA Extraction
Browse by: Latest US Patents, China's latest patents, Technical Efficacy Thesaurus, Application Domain, Technology Topic.
© 2024 PatSnap. All rights reserved.Legal|Privacy policy|Modern Slavery Act Transparency Statement|Sitemap