Session recommendation model fusing user microcosmic behaviors and knowledge graph
A knowledge graph and model technology, applied in biological neural network models, marketing, data processing applications, etc., can solve problems such as poor recommendation effect, neglect of microscopic behaviors of user-item interaction, and insufficient use of item knowledge to improve accuracy. Effect
- Summary
- Abstract
- Description
- Claims
- Application Information
AI Technical Summary
Problems solved by technology
Method used
Image
Examples
Embodiment Construction
[0070] The advantages of the present invention are further described below through specific implementation examples and comparative experiments.
[0071] 1. Hyperparameter settings. The MKM-SR model of the present invention and other comparison models all have the same embedding representation (vector) dimension, namely 100 dimensions. All embedding representations are initialized with a Gaussian distribution with zero mean and 0.1 standard deviation. Set the number of iterations of GGNN to 1. The multi-task learning of MKM-SR and its variant (weakened) model adopts an alternate training method. In addition, the experiment uses the Adam optimizer to learn the parameters in the model, the learning batch (batch-size) size is set to 128, and the weight λ of knowledge learning in formula 3 1 Set to 0.001.
[0072]2. Compare the experimental results and analysis. Based on two data sets KKBOX and JDATA to conduct comparative experiments, the evaluation indicators use Hit@k and ...
PUM
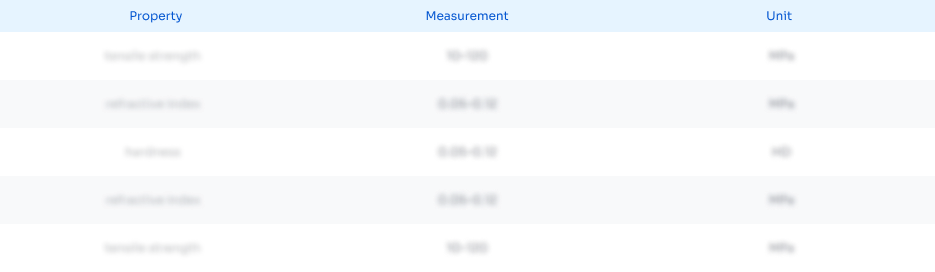
Abstract
Description
Claims
Application Information
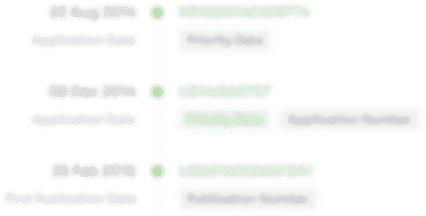
- R&D
- Intellectual Property
- Life Sciences
- Materials
- Tech Scout
- Unparalleled Data Quality
- Higher Quality Content
- 60% Fewer Hallucinations
Browse by: Latest US Patents, China's latest patents, Technical Efficacy Thesaurus, Application Domain, Technology Topic, Popular Technical Reports.
© 2025 PatSnap. All rights reserved.Legal|Privacy policy|Modern Slavery Act Transparency Statement|Sitemap|About US| Contact US: help@patsnap.com