Low-field-intensity MR stomach segmentation method based on transfer learning image enhancement
A transfer learning and image enhancement technology, applied in the field of medical image processing, can solve the problems of many artifacts, low field intensity MR images with large noise, and less image data, etc., to improve the segmentation performance, optimize the convolution structure, and avoid human factors. the effect of interference
- Summary
- Abstract
- Description
- Claims
- Application Information
AI Technical Summary
Problems solved by technology
Method used
Image
Examples
Embodiment Construction
[0046] The specific embodiments and effects of the present invention will be further described below in conjunction with the accompanying drawings.
[0047] refer to figure 1 , the low-field-strength MR gastric image segmentation method based on migration learning image enhancement proposed by the present invention comprises the following steps:
[0048] Step 1: Data Preparation:
[0049] Acquire high-field-strength 3DMR gastric image set A high And the low field strength 3DMR gastric image set A to be segmented low , respectively preprocessing them, preprocessing here refers to offset field correction, image resampling, image cropping and data normalization processing, etc., to obtain a preprocessed high field strength 3DMR stomach image set X and The low-field-strength 3DMR gastric image set Y to be segmented after preprocessing, and the resolution, size and grayscale interval of X and Y are consistent;
[0050] High field strength 3DMR gastric image set A high After bi...
PUM
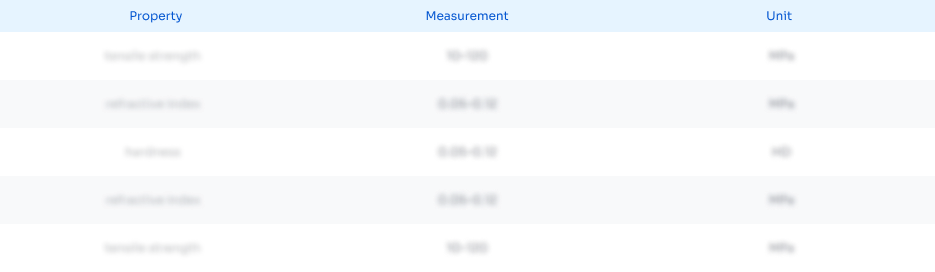
Abstract
Description
Claims
Application Information
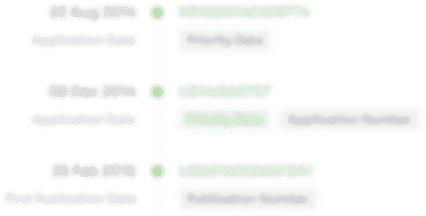
- R&D
- Intellectual Property
- Life Sciences
- Materials
- Tech Scout
- Unparalleled Data Quality
- Higher Quality Content
- 60% Fewer Hallucinations
Browse by: Latest US Patents, China's latest patents, Technical Efficacy Thesaurus, Application Domain, Technology Topic, Popular Technical Reports.
© 2025 PatSnap. All rights reserved.Legal|Privacy policy|Modern Slavery Act Transparency Statement|Sitemap|About US| Contact US: help@patsnap.com