Short-term scheduling rule extraction method based on variable structure deep learning framework
A technology of deep learning and extraction methods, applied in the direction of neural architecture, instruments, data processing applications, etc., can solve the problems of training sample data difficulties, scheduling results difficult to apply, etc., and achieve good decision support effects
- Summary
- Abstract
- Description
- Claims
- Application Information
AI Technical Summary
Problems solved by technology
Method used
Image
Examples
Embodiment Construction
[0028] The technical solutions in the present invention will be clearly and completely described below in conjunction with the accompanying drawings in the present invention.
[0029] figure 1 Shown is a schematic flow chart of one embodiment of the short-term scheduling rule extraction method based on the variable structure deep learning framework of the present invention, which specifically includes the following steps:
[0030] Step 1. Build a deep learning network: for the application scenario of the present invention, that is, how to extract the water level operation process of the power station on this day by using the incoming water sequence of the hydropower station, the initial and final control water level and the load sequence of the power grid, which can be regarded as a Typical black box problem. It is easy to know from the daily scheduling process of hydropower stations that this problem has time dependence, and the neural network has a strong ability to deal wi...
PUM
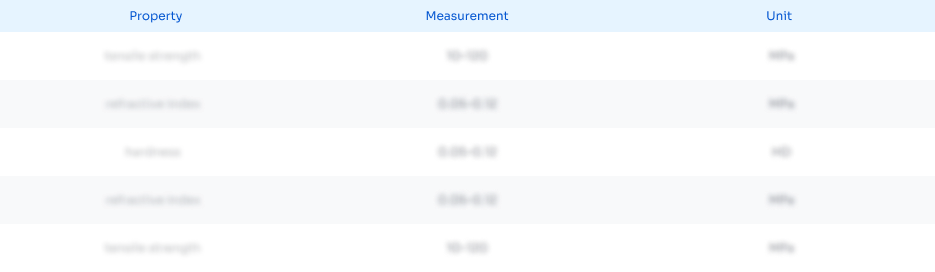
Abstract
Description
Claims
Application Information
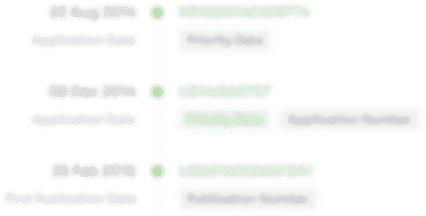
- R&D
- Intellectual Property
- Life Sciences
- Materials
- Tech Scout
- Unparalleled Data Quality
- Higher Quality Content
- 60% Fewer Hallucinations
Browse by: Latest US Patents, China's latest patents, Technical Efficacy Thesaurus, Application Domain, Technology Topic, Popular Technical Reports.
© 2025 PatSnap. All rights reserved.Legal|Privacy policy|Modern Slavery Act Transparency Statement|Sitemap|About US| Contact US: help@patsnap.com