Titanium alloy constitutive relation prediction method based on machine learning
A constitutive relationship and machine learning technology, applied in the field of material science and metal material constitutive behavior prediction, can solve problems such as data ambiguity, unseen metal material prediction, failure to meet data fitting accuracy requirements, etc.
- Summary
- Abstract
- Description
- Claims
- Application Information
AI Technical Summary
Problems solved by technology
Method used
Image
Examples
Embodiment 1
[0057] The specific steps for predicting the constitutive relationship of titanium alloys based on machine learning are as follows:
[0058] S01-1: Obtain the real stress-strain curves of 13 kinds of titanium alloys under room temperature (25°C) quasi-static compression, high-temperature quasi-static compression and room temperature dynamic compression test conditions, and record them together with the experimental conditions, a total of 135 pieces of data; Among them, for the test data under repeated conditions, all quasi-static compressive stress-strain curves are retained, while for high-temperature quasi-static compressive or room temperature dynamic compressive stress-strain curves, only one curve is retained under the same temperature or strain rate conditions, all grades The temperature range of the high temperature quasi-static compression test is between 300°C and 550°C, and the strain rate of the dynamic compression test at room temperature is 1400s -1 ~4200s -1 Amo...
PUM
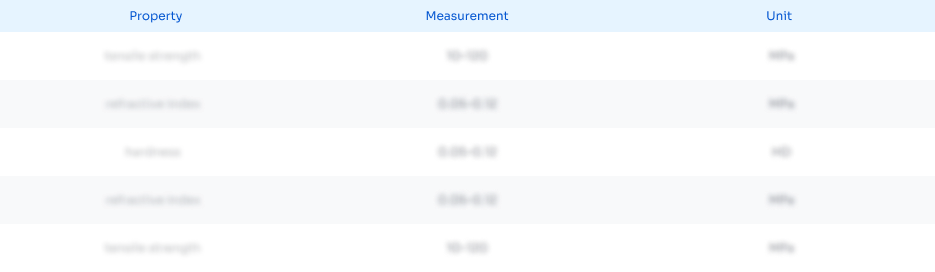
Abstract
Description
Claims
Application Information
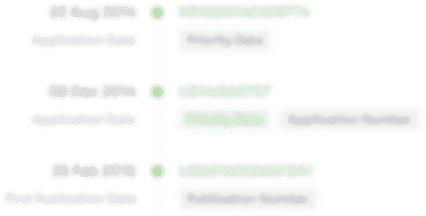
- R&D
- Intellectual Property
- Life Sciences
- Materials
- Tech Scout
- Unparalleled Data Quality
- Higher Quality Content
- 60% Fewer Hallucinations
Browse by: Latest US Patents, China's latest patents, Technical Efficacy Thesaurus, Application Domain, Technology Topic, Popular Technical Reports.
© 2025 PatSnap. All rights reserved.Legal|Privacy policy|Modern Slavery Act Transparency Statement|Sitemap|About US| Contact US: help@patsnap.com