Multi-modal process fault detection method based on weighted SVDD
A fault detection and multi-modal technology, applied in the direction of kernel methods, data processing applications, instruments, etc., can solve the problems that the design of weighting factors needs to be improved, rarely consider the nonlinear and non-Gaussian characteristics of multi-modal data, and achieve effective Conducive to modeling, sensitive identification, and avoiding the effect of overfitting
- Summary
- Abstract
- Description
- Claims
- Application Information
AI Technical Summary
Problems solved by technology
Method used
Image
Examples
Embodiment 1
[0063] A multimodal process fault detection method based on weighted SVDD, such as figure 1 As shown, including: offline modeling phase and fault detection phase;
[0064] The offline modeling phase includes:
[0065] Obtain normal data of different modalities to form an initial data set X, and use a sliding window of length w to slide on the initial data set X to obtain a window data sequence;
[0066] Calculate the statistics of each window data in the window data sequence, respectively as a sample, and calculate the local reachable density ratio of each sample respectively, as the weight factor corresponding to each sample, so as to use the weighted SVDD algorithm to establish a hypersphere model; The accessible density ratio is the normalized value of the local accessible density of the sample;
[0067] Optimizing the hypersphere model to obtain the Lagrangian factor α i , and the center a and radius R of the hypersphere; among them, 1≤i≤N, N represents the length of th...
Embodiment 2
[0122] A computer-readable storage medium includes a stored computer program. When the computer program is executed by a processor, the device where the computer-readable storage medium is located is controlled to execute the weighted SVDD-based multimodal process fault detection method provided in Embodiment 1 above.
[0123] The beneficial effects obtained by the present invention will be further explained in conjunction with specific application scenarios below.
[0124] In one of the application scenarios, 200 low-density banana-shaped two-dimensional samples are generated as mode 1, 100 high-density normal distribution two-dimensional samples are generated as mode 2, and 3 outliers close to high-density samples point. The global density and weighting factor of each sample are respectively as figure 2 and 3 As shown, the 1st to 200th sampling points are the sampling points of mode 1, the 201st to 300th sampling points are the sampling points of mode 2, and the 301st to ...
PUM
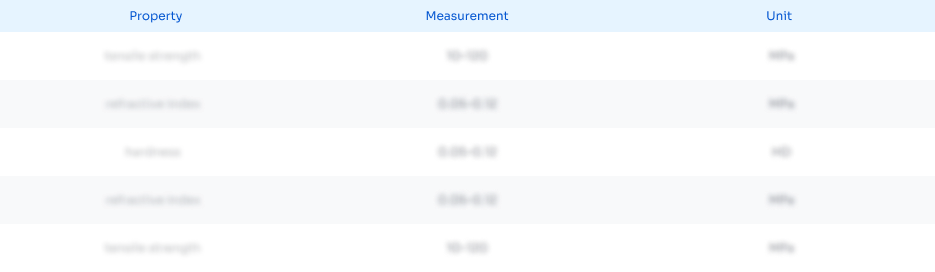
Abstract
Description
Claims
Application Information
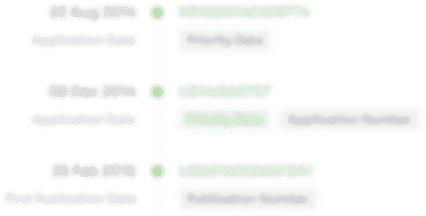
- R&D Engineer
- R&D Manager
- IP Professional
- Industry Leading Data Capabilities
- Powerful AI technology
- Patent DNA Extraction
Browse by: Latest US Patents, China's latest patents, Technical Efficacy Thesaurus, Application Domain, Technology Topic, Popular Technical Reports.
© 2024 PatSnap. All rights reserved.Legal|Privacy policy|Modern Slavery Act Transparency Statement|Sitemap|About US| Contact US: help@patsnap.com