Parallel multi-scale attention mechanism semantic segmentation method and device based on deep learning
A deep learning and semantic segmentation technology, applied in the field of deep learning and computer vision, can solve the problem of not taking into account the differences in different receptive fields, and achieve the effect of increasing the receptive field, improving the segmentation accuracy, and improving the accuracy.
- Summary
- Abstract
- Description
- Claims
- Application Information
AI Technical Summary
Problems solved by technology
Method used
Image
Examples
Embodiment Construction
[0067] The present embodiment will be further described below in conjunction with the accompanying drawings.
[0068] The image processing process involved in this embodiment is as follows: figure 1 shown in figure 1 The neural network model structure includes image preprocessing, downsampling feature extraction module, parallel multi-scale attention module, and upsampling model. The parallel multi-scale attention module includes the ASPP module and the EDS module.
[0069] The preprocessing stage can be understood as a kind of data enhancement, that is, rotating, scaling, cropping and flipping the image. By preprocessing the image, the semantic segmentation effect can be improved and the robustness of the model can be enhanced. Specifically, in this embodiment, the image is first randomly reduced or enlarged by a factor of 0.5 to 1.5, and corresponding padding is performed after reduction, or corresponding cropping is performed after enlargement, so that the image returns ...
PUM
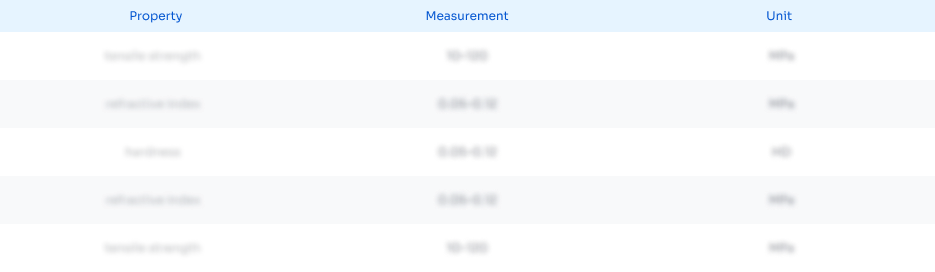
Abstract
Description
Claims
Application Information
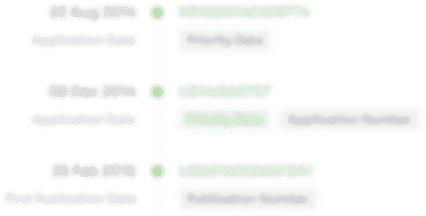
- R&D
- Intellectual Property
- Life Sciences
- Materials
- Tech Scout
- Unparalleled Data Quality
- Higher Quality Content
- 60% Fewer Hallucinations
Browse by: Latest US Patents, China's latest patents, Technical Efficacy Thesaurus, Application Domain, Technology Topic, Popular Technical Reports.
© 2025 PatSnap. All rights reserved.Legal|Privacy policy|Modern Slavery Act Transparency Statement|Sitemap|About US| Contact US: help@patsnap.com