Liver CT image multi-lesion classification method based on sample generation and transfer learning
A CT image and transfer learning technology, applied in the field of image processing, can solve the problems of detection and classification of multiple types of lesions, and achieve the effect of improving lesion classification performance, accurate and fast lesion classification performance, and speeding up training.
- Summary
- Abstract
- Description
- Claims
- Application Information
AI Technical Summary
Problems solved by technology
Method used
Image
Examples
Embodiment Construction
[0040] The present invention will be described in further detail below in conjunction with the accompanying drawings.
[0041] refer to figure 1 , the implementation steps of the present invention are as follows:
[0042] Step 1. Divide the dataset, mark the outline of the liver organ and store it.
[0043] 1.1) Extract 420 2D liver CT images from the liver CT images, and randomly select 80% of the images as a training set, and the remaining 20% as a test set;
[0044] 1.2) Mark the contours of liver organs on all 2D liver CT images and store them as mask images.
[0045] Step 2. Construct a liver organ segmentation network U based on global attention.
[0046] 2.1) Introduce the global attention upsampling GAU module in the existing segmentation network UNet:
[0047] The global attention upsampling GAU module, such as figure 2 As shown, it includes 3*3 convolutional layer, 1*1 convolutional layer, global pooling layer, input low-level feature map and high-level featu...
PUM
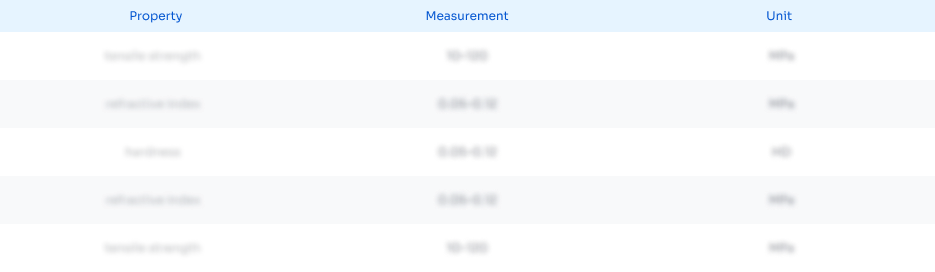
Abstract
Description
Claims
Application Information
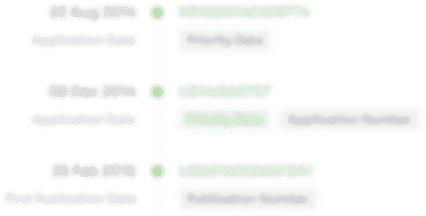
- R&D Engineer
- R&D Manager
- IP Professional
- Industry Leading Data Capabilities
- Powerful AI technology
- Patent DNA Extraction
Browse by: Latest US Patents, China's latest patents, Technical Efficacy Thesaurus, Application Domain, Technology Topic, Popular Technical Reports.
© 2024 PatSnap. All rights reserved.Legal|Privacy policy|Modern Slavery Act Transparency Statement|Sitemap|About US| Contact US: help@patsnap.com