Traffic prediction method based on enhanced space-time diagram neural network
A neural network and traffic prediction technology, applied in the field of traffic prediction based on the enhanced spatiotemporal graph neural network, can solve the problems of accumulation and differences in the next action, and achieve the effect of improving the prediction accuracy.
- Summary
- Abstract
- Description
- Claims
- Application Information
AI Technical Summary
Problems solved by technology
Method used
Image
Examples
Embodiment Construction
[0040] The following will clearly and completely describe the technical solutions in the embodiments of the present invention with reference to the drawings in the embodiments of the present invention. Based on the embodiments of the present invention, all other embodiments obtained by persons of ordinary skill in the art without making creative efforts belong to the protection scope of the present invention.
[0041] Such as figure 1 Shown, the present invention comprises the following steps,
[0042] S1: Preprocessing the raw traffic data collected by the sensor within a certain period of time;
[0043] The preprocessing process in the step S1 is to collect traffic data through sensors around the road at intervals of 5 minutes, extract the characteristics of the traffic data samples, process the original data through filtering and normalization, and eliminate invalid data to obtain Time series data; obtain the location information (longitude, latitude) of the sensor, and n...
PUM
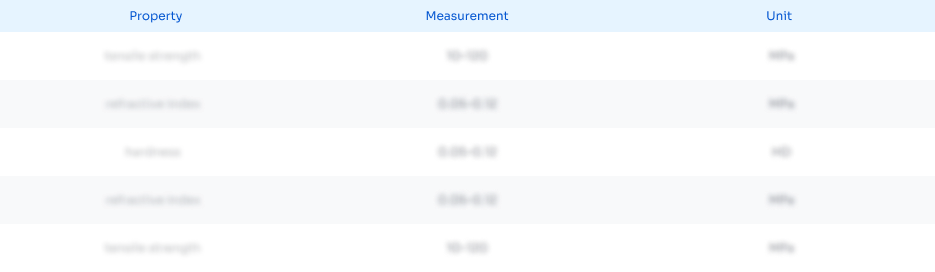
Abstract
Description
Claims
Application Information
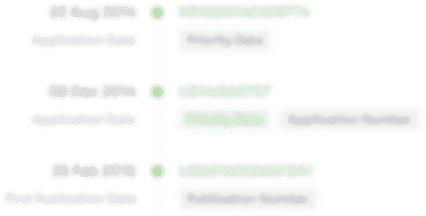
- R&D
- Intellectual Property
- Life Sciences
- Materials
- Tech Scout
- Unparalleled Data Quality
- Higher Quality Content
- 60% Fewer Hallucinations
Browse by: Latest US Patents, China's latest patents, Technical Efficacy Thesaurus, Application Domain, Technology Topic, Popular Technical Reports.
© 2025 PatSnap. All rights reserved.Legal|Privacy policy|Modern Slavery Act Transparency Statement|Sitemap|About US| Contact US: help@patsnap.com