Non-small cell lung cancer pathological section identification method based on deep convolutional neural network
A technology of non-small cell lung cancer and convolutional neural network, which is applied in the field of image processing, deep learning, and medical imaging to achieve good performance, improve network accuracy, and reduce the burden
- Summary
- Abstract
- Description
- Claims
- Application Information
AI Technical Summary
Problems solved by technology
Method used
Image
Examples
Embodiment Construction
[0049]The specific implementation of the present invention will be described in detail below in conjunction with the accompanying drawings. As a part of this specification, the principles of the present invention will be described through examples. Other aspects, features and advantages of the present invention will become clear through the detailed description. In the referenced drawings, the same reference numerals are used for the same or similar components in different drawings.
[0050] Such as Figure 1 to Figure 5 As shown, the non-small cell lung cancer pathological slice recognition method based on deep convolutional neural network provided by the present invention comprises the following steps:
[0051] S1: Preprocessing the pathological slice data of non-small cell lung cancer to be identified to obtain training set data and test set data.
[0052] Step S1 includes the following sub-steps:
[0053] S1.1: Since the pathological slices are too large, they are not su...
PUM
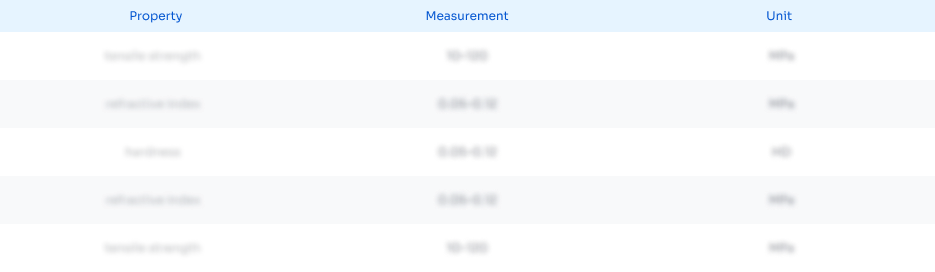
Abstract
Description
Claims
Application Information
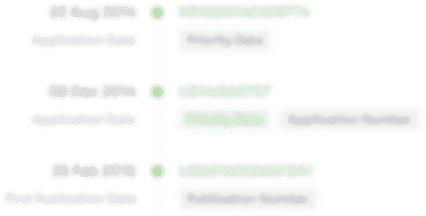
- R&D Engineer
- R&D Manager
- IP Professional
- Industry Leading Data Capabilities
- Powerful AI technology
- Patent DNA Extraction
Browse by: Latest US Patents, China's latest patents, Technical Efficacy Thesaurus, Application Domain, Technology Topic, Popular Technical Reports.
© 2024 PatSnap. All rights reserved.Legal|Privacy policy|Modern Slavery Act Transparency Statement|Sitemap|About US| Contact US: help@patsnap.com