Entity alignment method and device suitable for multi-modal knowledge graph
A knowledge graph, multi-modal technology, applied in the field of knowledge graph in natural language processing, can solve the problems of insufficient extraction of VGG model and limited alignment effectiveness.
- Summary
- Abstract
- Description
- Claims
- Application Information
AI Technical Summary
Problems solved by technology
Method used
Image
Examples
Embodiment 1
[0044] Such as figure 1 As shown, an entity alignment method suitable for multi-modal knowledge graphs includes the following steps:
[0045] Step 1, get two multimodal knowledge graphs and The data;
[0046] Step 2, project the data of each modality to the hyperbolic space;
[0047] Step 3, use the hyperbolic graph convolutional neural network to learn the structural features and visual features of the entity;
[0048] Step 4, fusing multimodal features;
[0049] Step 5, express entity similarity with distance in hyperbolic space;
[0050] Step 6, perform entity recognition alignment according to the similarity.
[0051] The entity alignment method of this embodiment, that is, the method operating in a hyperbolic space, will be described in detail below.
[0052] First, a hyperbolic graph convolutional neural network is adopted to learn the structural information of entities.
[0053] Then, the densenet model is used to convert the images associated with the entitie...
Embodiment 2
[0086] An entity alignment device suitable for multimodal knowledge graphs, including:
[0087] processor;
[0088] And, a memory for storing executable instructions of the processor;
[0089] Wherein, the processor is configured to execute the above entity alignment method by executing the executable instruction in the first embodiment.
[0090] Those skilled in the art should understand that the embodiments of the present application may be provided as methods, systems or computer program products. Accordingly, the present application can take the form of an entirely hardware embodiment, an entirely software embodiment or an embodiment combining software and hardware aspects. Furthermore, the present application may take the form of a computer program product embodied on one or more computer-usable storage media (including but not limited to disk storage, CD-ROM, optical storage, etc.) having computer-usable program code embodied therein.
PUM
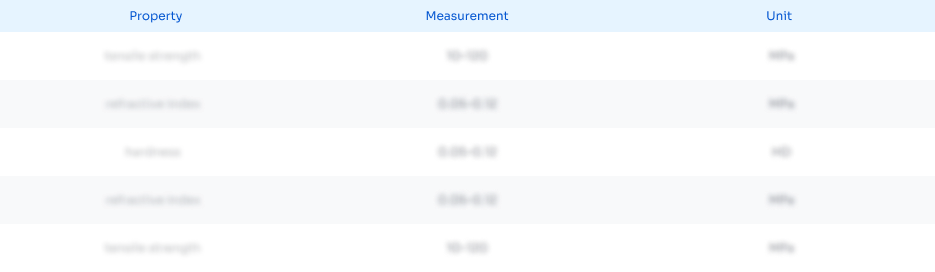
Abstract
Description
Claims
Application Information
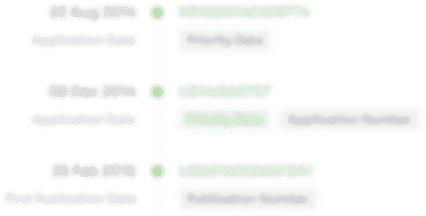
- R&D Engineer
- R&D Manager
- IP Professional
- Industry Leading Data Capabilities
- Powerful AI technology
- Patent DNA Extraction
Browse by: Latest US Patents, China's latest patents, Technical Efficacy Thesaurus, Application Domain, Technology Topic, Popular Technical Reports.
© 2024 PatSnap. All rights reserved.Legal|Privacy policy|Modern Slavery Act Transparency Statement|Sitemap|About US| Contact US: help@patsnap.com