Method for improving brain-computer interface performance based on dynamic inverse learning network
A technology of learning network and brain-computer interface, which is applied in the field of improving the performance of brain-computer interface based on dynamic inverse learning network, can solve the problems of over-fitting of classifier variability, signal distortion, low signal-to-noise ratio, etc., and achieve good convergence , the effect of fast convergence speed
- Summary
- Abstract
- Description
- Claims
- Application Information
AI Technical Summary
Problems solved by technology
Method used
Image
Examples
Embodiment
[0063] Figure 1a and Figure 1b Shown is the user interface of the P300 speller. In this embodiment, the user interface consists of 36 characters (6×6 matrix). The spelling principle is described as follows: The position of the character is determined by the intersection of the row and column of the matrix. Users always focus on the characters they need. In this process, all the rows and columns of the character matrix are randomly lit sequentially. A P300 signal is generated due to visual stimulation when the desired character row or column is illuminated. By detecting the user's P300 signal, the position of the desired character can be obtained. For an epoch or round, there are 12 flashes (one row or one column at a time, Scrabble has six rows and six columns), and only two of these rows are needed for the desired character. An epoch repeats 15 times. In addition, each blink means that a single row or column is lit for 100ms and blank for 75ms. Acquired by a 64-channe...
PUM
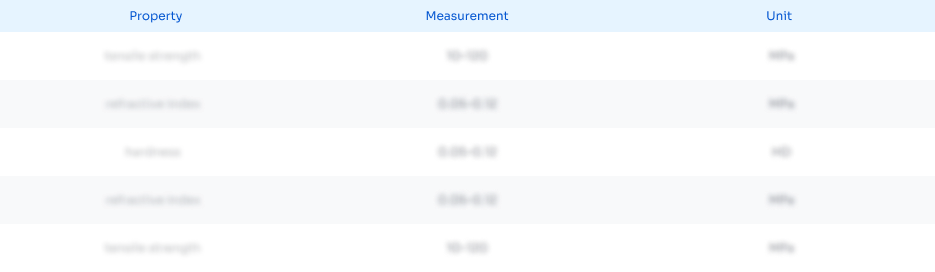
Abstract
Description
Claims
Application Information
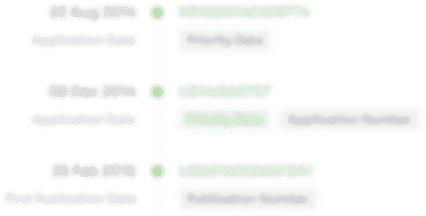
- R&D Engineer
- R&D Manager
- IP Professional
- Industry Leading Data Capabilities
- Powerful AI technology
- Patent DNA Extraction
Browse by: Latest US Patents, China's latest patents, Technical Efficacy Thesaurus, Application Domain, Technology Topic.
© 2024 PatSnap. All rights reserved.Legal|Privacy policy|Modern Slavery Act Transparency Statement|Sitemap