Context-aware multi-view three-dimensional reconstruction system and method based on deep learning
A 3D reconstruction and deep learning technology, applied in the deep learning-based context-aware multi-view 3D reconstruction system and its field, can solve problems such as inability to make full use of input images, inconsistent 3D shapes, and inability to process in parallel, achieving high consistency, The effect of shape optimization stabilization and faster reconstruction
- Summary
- Abstract
- Description
- Claims
- Application Information
AI Technical Summary
Problems solved by technology
Method used
Image
Examples
Embodiment Construction
[0039] A deep learning-based context-based context Figure three Dimensional redding system and methods, including encoders, decoders, context fusion modules, refiners, and corresponding network loss functions. The encoder generates n feature map according to the N input images; the decoder is used as input, and the N initial three-dimensional shape is reconstructed; the context fusion module uses the initial three-dimensional shape as input, and adaptively selects each The initial three-dimensional shape of the mass is fused to obtain a three-dimensional shape of the fusion; the seminulator will fuse the three-dimensional shape as input, further correct the reconstructed error portion, and then reconstruct the final three-dimensional shape. The present invention provides a technique for rapid reconstructing objects in three-dimensional shapes in a view that is visually seen from a plurality of angles.
[0040] The present invention will be further described in detail below with r...
PUM
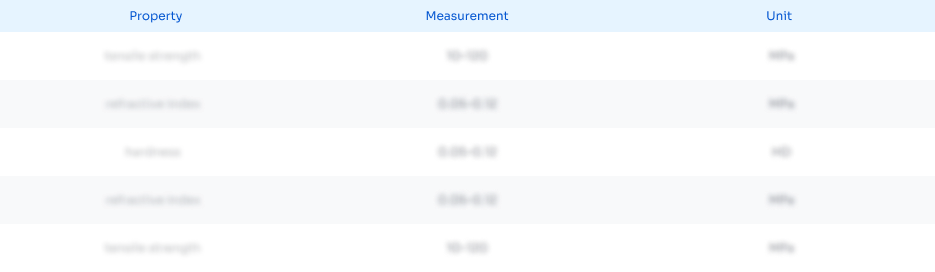
Abstract
Description
Claims
Application Information
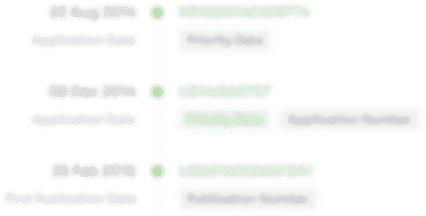
- R&D
- Intellectual Property
- Life Sciences
- Materials
- Tech Scout
- Unparalleled Data Quality
- Higher Quality Content
- 60% Fewer Hallucinations
Browse by: Latest US Patents, China's latest patents, Technical Efficacy Thesaurus, Application Domain, Technology Topic, Popular Technical Reports.
© 2025 PatSnap. All rights reserved.Legal|Privacy policy|Modern Slavery Act Transparency Statement|Sitemap|About US| Contact US: help@patsnap.com