Dynamic-to-static scene conversion method based on conditional generative adversarial network
A technology of conditional generation and scene conversion, applied in biological neural network models, computer components, instruments, etc., can solve the problems of poor performance in image texture and details, achieve optimized semantic consistency, improve authenticity, and improve stability Effect
- Summary
- Abstract
- Description
- Claims
- Application Information
AI Technical Summary
Problems solved by technology
Method used
Image
Examples
Embodiment 1
[0014] Embodiment 1: see figure 1 , a dynamic-to-static scene conversion method based on a conditional generative confrontation network, including the following steps:
[0015] Step 1: Data preprocessing stage;
[0016] Step 2: Model construction phase, the model includes a cascaded two-stage generation network from coarse to fine, and two types of discriminant networks,
[0017] Step 3: Model parameter training phase,
[0018] Step 4: Dynamic to static scene image conversion module stage;
[0019] In the data preprocessing stage, the data is processed to meet the requirements of the network. Firstly, the images in the data set are randomly cropped and scaled to make training data, and multiple sets of training data are constructed using the dynamic scene at the same location, the binary mask of the dynamic target, and the static scene as a set of data.
[0020] In the model building phase, the corresponding deep neural network is designed and built according to the model....
PUM
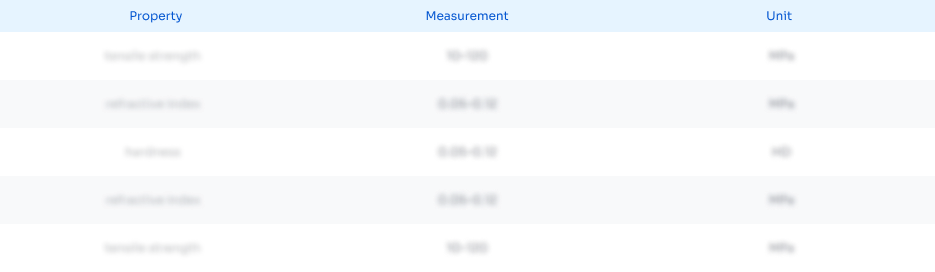
Abstract
Description
Claims
Application Information
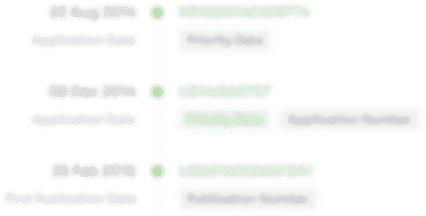
- R&D Engineer
- R&D Manager
- IP Professional
- Industry Leading Data Capabilities
- Powerful AI technology
- Patent DNA Extraction
Browse by: Latest US Patents, China's latest patents, Technical Efficacy Thesaurus, Application Domain, Technology Topic, Popular Technical Reports.
© 2024 PatSnap. All rights reserved.Legal|Privacy policy|Modern Slavery Act Transparency Statement|Sitemap|About US| Contact US: help@patsnap.com