Signal semantic recognition method based on multistage semantic representation and semantic calculation
A semantic recognition and semantic computing technology, applied in character and pattern recognition, computing, computer components, etc., can solve problems such as insufficient generalization ability, poor interpretability, and difficulty in migration
- Summary
- Abstract
- Description
- Claims
- Application Information
AI Technical Summary
Problems solved by technology
Method used
Image
Examples
Embodiment Construction
[0032] The present invention will be further described in detail below in conjunction with the accompanying drawings and specific embodiments.
[0033] refer to figure 1 , the implementation steps of this example are as follows:
[0034] Step 1, obtain training sample set and test sample set.
[0035]Obtain a training sample set and a test sample set from the signal semantic recognition dataset. Existing signal semantic recognition datasets include MNIST handwritten digit dataset, CIFAR dataset, and ImageNet dataset. In this example, the MNIST handwritten digit recognition dataset is preferred but not limited to the signal semantic recognition dataset. The MNIST handwritten digit recognition dataset contains 70,000 single-channel handwritten digit image samples of size 28×28 and 70,000 one-hot label vectors of length 10;
[0036] Randomly select M=60,000 labeled image signals from the MNIST handwritten digit recognition dataset to form a training sample set S a , and the r...
PUM
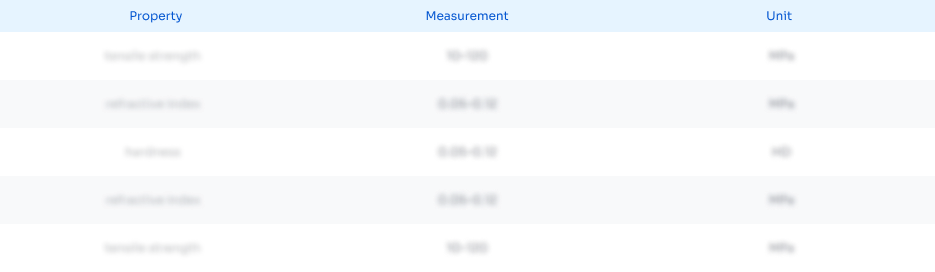
Abstract
Description
Claims
Application Information
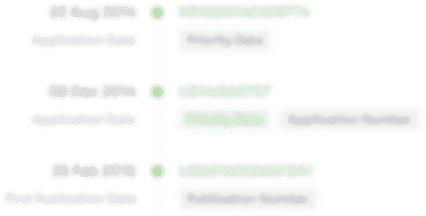
- R&D Engineer
- R&D Manager
- IP Professional
- Industry Leading Data Capabilities
- Powerful AI technology
- Patent DNA Extraction
Browse by: Latest US Patents, China's latest patents, Technical Efficacy Thesaurus, Application Domain, Technology Topic.
© 2024 PatSnap. All rights reserved.Legal|Privacy policy|Modern Slavery Act Transparency Statement|Sitemap