Few-sample data amplification method for target detection
A technology of target detection and sample data, which is applied in the field of target detection, can solve the problems that the algorithm is difficult to implement, the relationship between different target samples cannot be changed, and the diversity of target sample data cannot be changed, so as to achieve the effect of improving efficiency and data quality
- Summary
- Abstract
- Description
- Claims
- Application Information
AI Technical Summary
Problems solved by technology
Method used
Image
Examples
Embodiment Construction
[0026] In order to make the purpose, technical solutions and advantages of the present invention clearer, the present invention will be further described in detail below in conjunction with the examples and accompanying drawings. As a limitation of the present invention.
[0027] Such as figure 1 As shown, the few-sample data amplification method for target detection disclosed by the present invention comprises the following steps:
[0028] S1, qualitatively analyze the possible scenes and scene styles of the target sample;
[0029] S2, using the scene detection algorithm and the style extraction algorithm to extract the scene features and style features of the target sample;
[0030] S3, using machine learning algorithms to perform cluster analysis on scene features and style features;
[0031] S4, search for pictures with similar scenes or styles from the open source data set according to the cluster analysis results;
[0032] S5, fusing the target sample with similar pi...
PUM
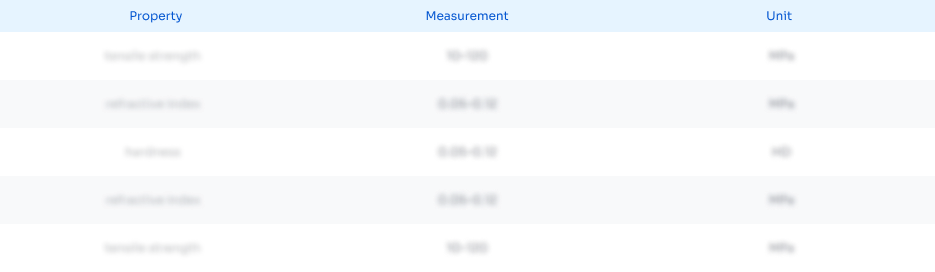
Abstract
Description
Claims
Application Information
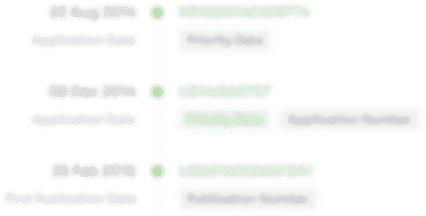
- R&D Engineer
- R&D Manager
- IP Professional
- Industry Leading Data Capabilities
- Powerful AI technology
- Patent DNA Extraction
Browse by: Latest US Patents, China's latest patents, Technical Efficacy Thesaurus, Application Domain, Technology Topic, Popular Technical Reports.
© 2024 PatSnap. All rights reserved.Legal|Privacy policy|Modern Slavery Act Transparency Statement|Sitemap|About US| Contact US: help@patsnap.com