Disease recognitionmethod based on lightweight twin convolutional neural network
A technology of convolutional neural network and recognition method, applied in the field of disease recognition based on lightweight twin convolutional neural network, can solve the problems of limited effective change mode of new image samples, risk of model fitting, complexity, etc., and achieve alleviation Incompatibility, enhancing feature discrimination ability, alleviating the effect of small differences between classes
- Summary
- Abstract
- Description
- Claims
- Application Information
AI Technical Summary
Problems solved by technology
Method used
Image
Examples
Embodiment Construction
[0034] The specific implementation of the present invention will be described in further detail below by describing the embodiments with reference to the accompanying drawings, so as to help those skilled in the art have a more complete, accurate and in-depth understanding of the inventive concepts and technical solutions of the present invention.
[0035] figure 1 The flow chart of the disease identification method based on the lightweight twin convolutional neural network provided by the embodiment of the present invention can be used for lesion identification of skin diseases. The method specifically includes the following steps:
[0036] The technical solution includes four processes, namely data set preprocessing, model construction and model training, and lesion identification and visualization.
[0037] 1) The process of data set preprocessing:
[0038]1) Obtain the ISIC2019 dermoscopic image database (the ISIC2019 database has 9 lesion categories, a total of 2.3W imag...
PUM
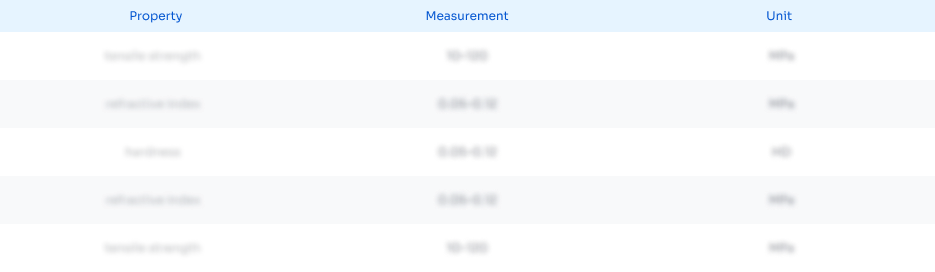
Abstract
Description
Claims
Application Information
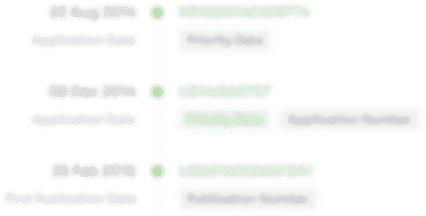
- R&D Engineer
- R&D Manager
- IP Professional
- Industry Leading Data Capabilities
- Powerful AI technology
- Patent DNA Extraction
Browse by: Latest US Patents, China's latest patents, Technical Efficacy Thesaurus, Application Domain, Technology Topic, Popular Technical Reports.
© 2024 PatSnap. All rights reserved.Legal|Privacy policy|Modern Slavery Act Transparency Statement|Sitemap|About US| Contact US: help@patsnap.com