Spike detection method based on temporal features and stacked bi-LSTM networks
A detection method and timing technology, applied in diagnostic recording/measurement, medical science, diagnosis, etc., can solve problems such as high computational complexity, harsh characterization ability, and ineffective use of EEG timing signals
- Summary
- Abstract
- Description
- Claims
- Application Information
AI Technical Summary
Problems solved by technology
Method used
Image
Examples
Embodiment Construction
[0070] The present invention will be described in detail below in conjunction with the accompanying drawings and specific embodiments.
[0071] Such as figure 1 As shown, the general implementation steps of the multi-channel joint detection method for spike discharge have been introduced in detail in the content of the invention, that is, the technical solution of the present invention mainly includes the following steps:
[0072] Step 1. Perform a preprocessing operation on the input pre-marked original single-channel EEG signal, the pre-marking is to mark the spike and non-spike time points of the original single-channel EEG signal; the preprocessing The operation includes cascade filtering and normalization processing; finally, according to the duration characteristics of the detection target waveform, the preprocessed EEG signal is segmented in the time domain to obtain EEG signal fragments, and data enhancement is performed on the training set spike data.
[0073] Step 2...
PUM
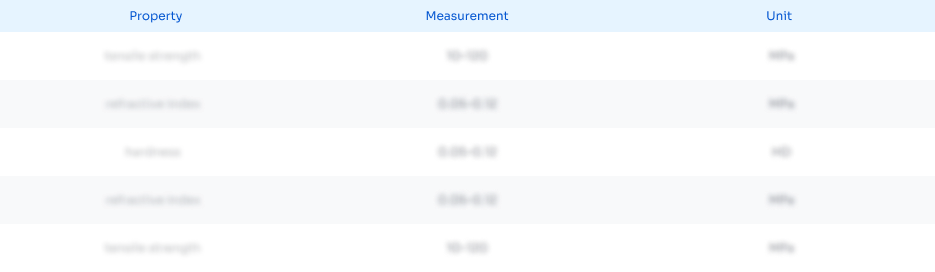
Abstract
Description
Claims
Application Information
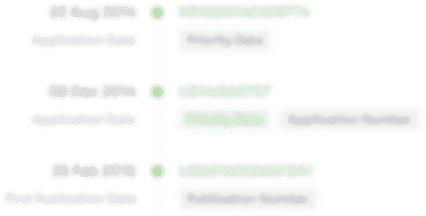
- R&D Engineer
- R&D Manager
- IP Professional
- Industry Leading Data Capabilities
- Powerful AI technology
- Patent DNA Extraction
Browse by: Latest US Patents, China's latest patents, Technical Efficacy Thesaurus, Application Domain, Technology Topic, Popular Technical Reports.
© 2024 PatSnap. All rights reserved.Legal|Privacy policy|Modern Slavery Act Transparency Statement|Sitemap|About US| Contact US: help@patsnap.com