Convolutional neural network weight gradient optimization method based on data stream
A convolutional neural network and gradient optimization technology, which is applied in the field of convolutional neural network training, can solve problems such as time-consuming, storage resource consumption, and transmission time, and achieve obvious acceleration effects, speed up network training, and reduce transmission time.
- Summary
- Abstract
- Description
- Claims
- Application Information
AI Technical Summary
Problems solved by technology
Method used
Image
Examples
Embodiment Construction
[0027] The technical solutions of the present invention will be described in detail below in conjunction with the accompanying drawings and embodiments.
[0028] The weight gradient optimization calculation method of the convolutional neural network based on the data flow mode of the present invention optimizes the weight gradient. According to the formula, the gradient of the input image and the output image has a fixed difference (K-1). Such as image 3 As shown, it is a schematic diagram of a weight gradient optimization model of a convolutional neural network weight gradient optimization calculation method based on data flow in the present invention. The model slides the input image through a K×K sliding window, and changes the original unfixed convolution size to a fixed size K×K, which can support the calculation of weight gradients of any size. The specific calculation method is as follows: 1) Simultaneously calculate the input image covered by the first K×K window an...
PUM
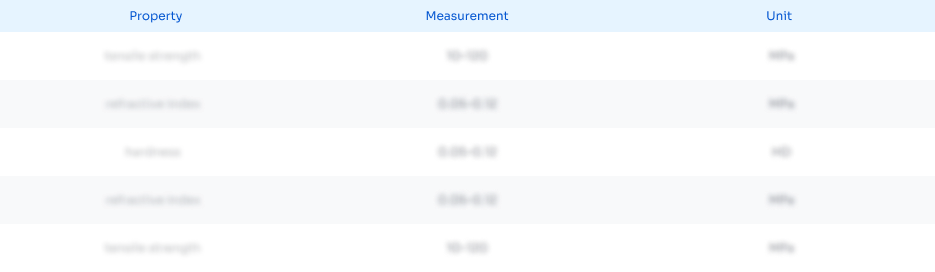
Abstract
Description
Claims
Application Information
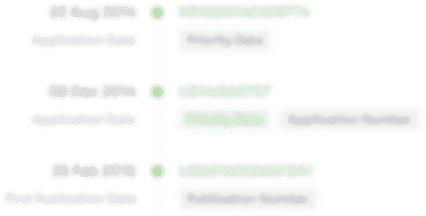
- R&D Engineer
- R&D Manager
- IP Professional
- Industry Leading Data Capabilities
- Powerful AI technology
- Patent DNA Extraction
Browse by: Latest US Patents, China's latest patents, Technical Efficacy Thesaurus, Application Domain, Technology Topic, Popular Technical Reports.
© 2024 PatSnap. All rights reserved.Legal|Privacy policy|Modern Slavery Act Transparency Statement|Sitemap|About US| Contact US: help@patsnap.com