Attention enhancement out-of-distribution image detection method based on classifier prediction uncertainty
An uncertainty, image detection technology, applied in the direction of instruments, neural learning methods, biological neural network models, etc., can solve the problems of large coverage of out-of-distribution data, unable to give reliable results, and not very good results, and achieve classification The results are reliable, the detection effect is improved, and the detection effect is good.
- Summary
- Abstract
- Description
- Claims
- Application Information
AI Technical Summary
Problems solved by technology
Method used
Image
Examples
Embodiment Construction
[0032] The accompanying drawings are for illustrative purposes only and cannot be construed as limiting the patent;
[0033] In order to better illustrate this embodiment, some parts in the drawings will be omitted, enlarged or reduced, and do not represent the size of the actual product;
[0034] For those skilled in the art, it is understandable that some well-known structures and descriptions thereof may be omitted in the drawings.
[0035] The technical solutions of the present invention will be further described below in conjunction with the accompanying drawings and embodiments.
[0036] Such as Figure 1-2 As shown, an attention-enhanced out-of-distribution image detection method based on classifier prediction uncertainty includes the following steps:
[0037] S1: Image reconstruction feature extraction and dimensionality reduction processing;
[0038] S2: Use the low-dimensional data obtained in S1 to perform multi-classification probability calculations, and extrac...
PUM
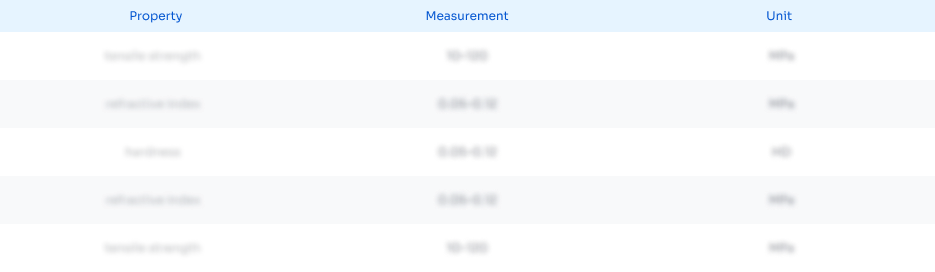
Abstract
Description
Claims
Application Information
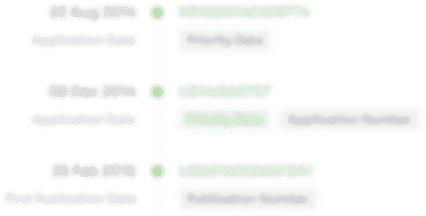
- R&D Engineer
- R&D Manager
- IP Professional
- Industry Leading Data Capabilities
- Powerful AI technology
- Patent DNA Extraction
Browse by: Latest US Patents, China's latest patents, Technical Efficacy Thesaurus, Application Domain, Technology Topic, Popular Technical Reports.
© 2024 PatSnap. All rights reserved.Legal|Privacy policy|Modern Slavery Act Transparency Statement|Sitemap|About US| Contact US: help@patsnap.com