No-reference video quality evaluation method based on deep spatio-temporal information
A technology of video quality and evaluation method, which is applied in video processing and image fields, and can solve the problems that the time memory model does not consider the frame rate, the result is inaccurate, and the information is not comprehensive enough.
- Summary
- Abstract
- Description
- Claims
- Application Information
AI Technical Summary
Problems solved by technology
Method used
Image
Examples
Embodiment Construction
[0066] Specific embodiments of the present invention will be described in detail below in conjunction with the accompanying drawings. It should be understood that the specific embodiments described here are only used to illustrate and explain the present invention, and are not intended to limit the present invention.
[0067] Such as Figure 1-4 As shown, the focus of the no-reference video quality assessment method based on deep spatiotemporal information is the quality assessment problem of real videos. Since humans are end users, leveraging knowledge of the Human Visual System (HVS) can help build objective approaches to our problems. Specifically, human perception of video quality is mainly influenced by single-frame image content and short-term memory.
[0068] The present invention is mainly divided into the following modules: content perception feature extraction and time memory model. Among them, the content-aware feature extraction module uses the Resnst-50 pre-tra...
PUM
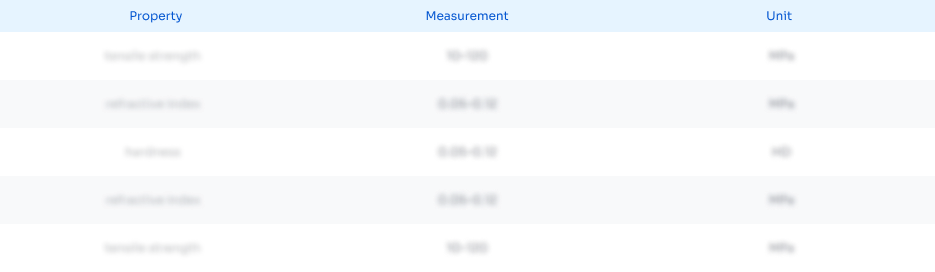
Abstract
Description
Claims
Application Information
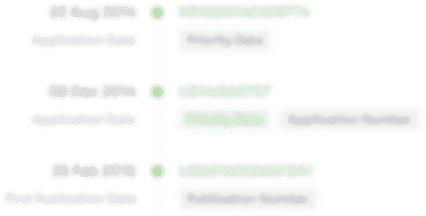
- Generate Ideas
- Intellectual Property
- Life Sciences
- Materials
- Tech Scout
- Unparalleled Data Quality
- Higher Quality Content
- 60% Fewer Hallucinations
Browse by: Latest US Patents, China's latest patents, Technical Efficacy Thesaurus, Application Domain, Technology Topic, Popular Technical Reports.
© 2025 PatSnap. All rights reserved.Legal|Privacy policy|Modern Slavery Act Transparency Statement|Sitemap|About US| Contact US: help@patsnap.com