Self-adaptive asynchronous federated learning method with local privacy protection
A privacy-preserving, learning-method technology, applied in the field of adaptive asynchronous federated learning
- Summary
- Abstract
- Description
- Claims
- Application Information
AI Technical Summary
Problems solved by technology
Method used
Image
Examples
Embodiment Construction
[0033] The present invention will be described in further detail below in conjunction with the accompanying drawings.
[0034] refer to figure 1 and figure 2 , the self-adaptive asynchronous federated learning method with local privacy protection provided by the present invention, the central server is responsible for the initialization of model parameters and system parameters and parameter transmission, the reception and aggregation of the user's local update amount, gradient clipping standard, noise variance, learning rate Adaptive adjustment, update of the global model, the user is responsible for the local gradient calculation, cropping, noise addition and sending of the global model. Specifically include the following steps:
[0035] 1) Parameter initialization: reference figure 2 , the central server establishes the training model, and is responsible for sending model parameters and system parameters, receiving user local updates, aggregation, and updating the glob...
PUM
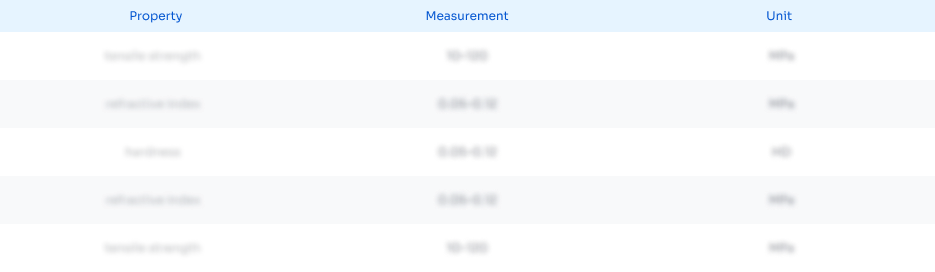
Abstract
Description
Claims
Application Information
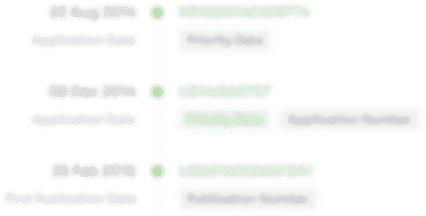
- R&D Engineer
- R&D Manager
- IP Professional
- Industry Leading Data Capabilities
- Powerful AI technology
- Patent DNA Extraction
Browse by: Latest US Patents, China's latest patents, Technical Efficacy Thesaurus, Application Domain, Technology Topic.
© 2024 PatSnap. All rights reserved.Legal|Privacy policy|Modern Slavery Act Transparency Statement|Sitemap