Aerial photography target tracking method fusing target saliency and online learning interference factors
A technology for learning interference and targets, applied in the field of computer vision, to achieve reliable adaptive matching tracking, effective and reliable adaptive matching tracking, and speed up tracking
- Summary
- Abstract
- Description
- Claims
- Application Information
AI Technical Summary
Problems solved by technology
Method used
Image
Examples
Embodiment Construction
[0058] In this embodiment, an aerial photography target tracking method that combines target saliency and online learning interference factors, such as figure 1 As shown, proceed as follows:
[0059] Step 1. Pre-train the general features of the fully convolutional twin network;
[0060] In this embodiment, a fully convolutional twin network is used, including 5 convolutional layers and 2 pooling layers, and each convolutional layer is followed by a normalization layer and an activation function layer;
[0061] Step 1.1. Obtain a tagged aerial data set. The aerial data set contains multiple video sequences, and each video sequence contains multiple frames. Select a video sequence and extract any i-th frame image and adjacent T frames in the current video sequence. Any frame of image in the image forms a sample pair, so that the randomly selected images in the current video sequence form several sample pairs, and then constitute the training data set;
[0062] Step 1.2, using...
PUM
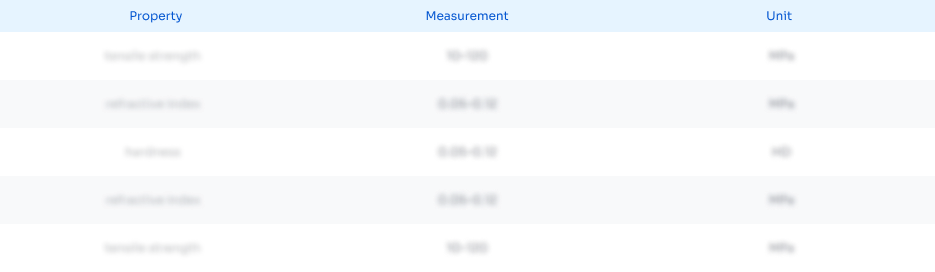
Abstract
Description
Claims
Application Information
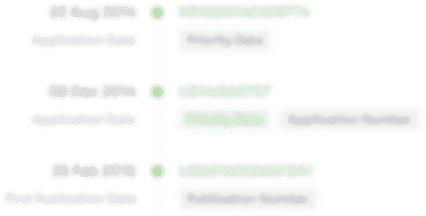
- R&D
- Intellectual Property
- Life Sciences
- Materials
- Tech Scout
- Unparalleled Data Quality
- Higher Quality Content
- 60% Fewer Hallucinations
Browse by: Latest US Patents, China's latest patents, Technical Efficacy Thesaurus, Application Domain, Technology Topic, Popular Technical Reports.
© 2025 PatSnap. All rights reserved.Legal|Privacy policy|Modern Slavery Act Transparency Statement|Sitemap|About US| Contact US: help@patsnap.com