Radio and television news keyword automatic extraction method based on deep learning
A technology of radio and television and deep learning, applied in neural learning methods, electrical digital data processing, natural language data processing, etc., can solve problems such as inability to obtain vocabulary and incomplete coverage, achieve accurate organization and management, and improve management efficiency Effect
- Summary
- Abstract
- Description
- Claims
- Application Information
AI Technical Summary
Problems solved by technology
Method used
Image
Examples
Embodiment 1
[0047] Such as figure 1 As shown, the automatic extraction method of radio and television news keywords based on deep learning includes steps:
[0048] S1, mark the keyword information of the radio and television news data to be analyzed, and construct a keyword data set;
[0049] S2, using the pre-training model to build a keyword extraction model, and using the keyword data set training in step S1 to build the keyword extraction model;
[0050] S3, using the keyword extraction model trained in step S2 to predict the input radio and television news, and obtain a keyword extraction result.
Embodiment 2
[0052] On the basis of Embodiment 1, the following steps are performed to construct the keyword data set in step S1:
[0053] S11, collect radio and television news data, and use the keyword results given by relevant professionals as candidate keywords; then clean the candidate keywords, remove meaningless and redundant keywords, and obtain the final keyword results; cleaning includes : First use entity recognition technology to identify entities in news text data, and remove entity words from candidate keywords; remove keywords that are too long or too short and keywords that do not appear in the original text. In this way, meaningless and redundant keywords can be removed, so that the features of meaningful keywords can be better identified in the subsequent training model, so that the trained model can better extract meaningful keywords.
[0054] S12, after the sentence and paragraph aggregation of the radio and television news text data, according to the final keyword resu...
Embodiment 3
[0056] On the basis of Embodiment 1, in step S2, the keyword extraction model includes a text vectorization layer, a first keyword prediction layer and a second keyword sequence labeling layer in series order.
[0057] The text vectorization layer adopts the pre-trained BERT layer to convert the text sequence Convert to a sequence of vectors ;in, represents the input text sequence, Represents the text vector sequence encoded by the text vector layer, and n represents the total number of characters in the input text sequence. In this embodiment, with the help of BERT's powerful language representation capabilities, better character-level semantic embedding expressions can be obtained.
[0058] The construction process of the first keyword prediction layer is as follows:
[0059] S21, for forward LSTM, define the bias parameters of the forget gate matrix and the forget gate matrix , the memory gate matrix and the bias parameter of the memory gate matrix , the outp...
PUM
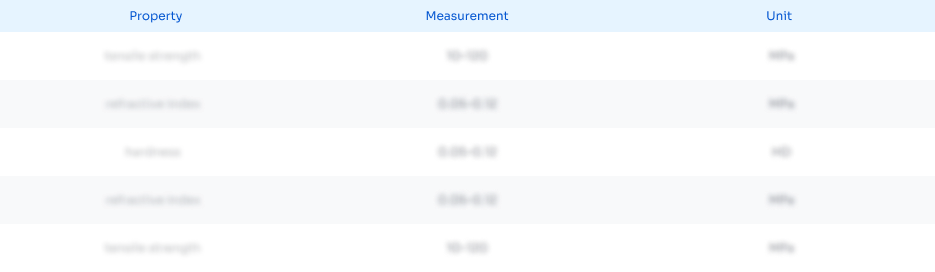
Abstract
Description
Claims
Application Information
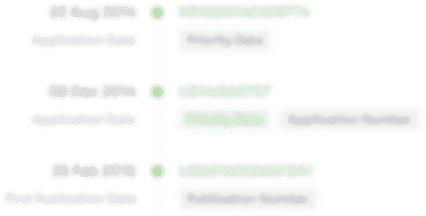
- R&D Engineer
- R&D Manager
- IP Professional
- Industry Leading Data Capabilities
- Powerful AI technology
- Patent DNA Extraction
Browse by: Latest US Patents, China's latest patents, Technical Efficacy Thesaurus, Application Domain, Technology Topic, Popular Technical Reports.
© 2024 PatSnap. All rights reserved.Legal|Privacy policy|Modern Slavery Act Transparency Statement|Sitemap|About US| Contact US: help@patsnap.com