Landslide displacement prediction method based on SVR-LSTM mixed deep learning
A prediction method and deep learning technology, applied in neural learning methods, prediction, biological neural network models, etc., can solve the problems of time-consuming and labor-intensive statistical models, low data usage, and low accuracy, so as to improve displacement prediction accuracy and reduce Modeling Cost, Stability, and Accuracy Guaranteed Effects
- Summary
- Abstract
- Description
- Claims
- Application Information
AI Technical Summary
Problems solved by technology
Method used
Image
Examples
Embodiment Construction
[0051] The present invention will be described in detail below in conjunction with the accompanying drawings and specific embodiments. But it does not limit the present invention.
[0052] Such as figure 1 As shown, the landslide displacement prediction method based on SVR-LSTM hybrid deep learning provided in this embodiment specifically includes the following steps:
[0053] Step 1: Collect landslide displacement data, and perform EMD decomposition on the displacement data to obtain multiple IMF components and residual items, and use IMF components as periodic items and residual items as trend items.
[0054] In this embodiment, 72 groups of landslide displacement data are obtained from the landslide mountain displacement monitoring points and influencing factor sensors, including displacement data, precipitation, maximum monthly precipitation, and water level inventory.
[0055] see figure 2 , IMF1 and IMF2 will be obtained as periodic items, and residual items as trend...
PUM
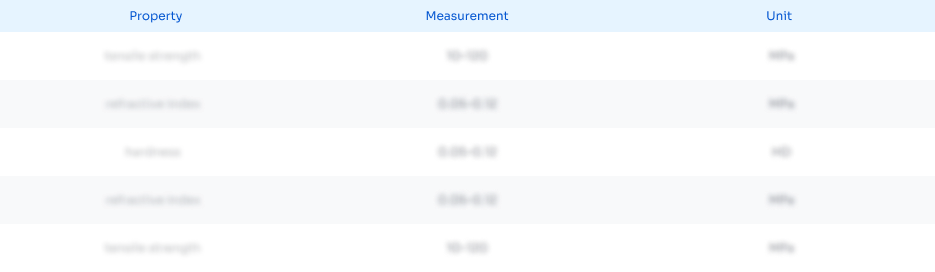
Abstract
Description
Claims
Application Information
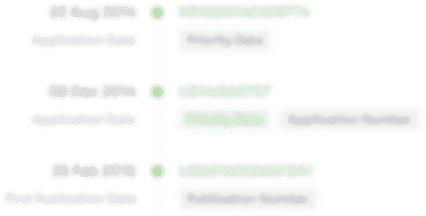
- R&D Engineer
- R&D Manager
- IP Professional
- Industry Leading Data Capabilities
- Powerful AI technology
- Patent DNA Extraction
Browse by: Latest US Patents, China's latest patents, Technical Efficacy Thesaurus, Application Domain, Technology Topic.
© 2024 PatSnap. All rights reserved.Legal|Privacy policy|Modern Slavery Act Transparency Statement|Sitemap